Self-supervised Representation Learning for Autonomous Driving
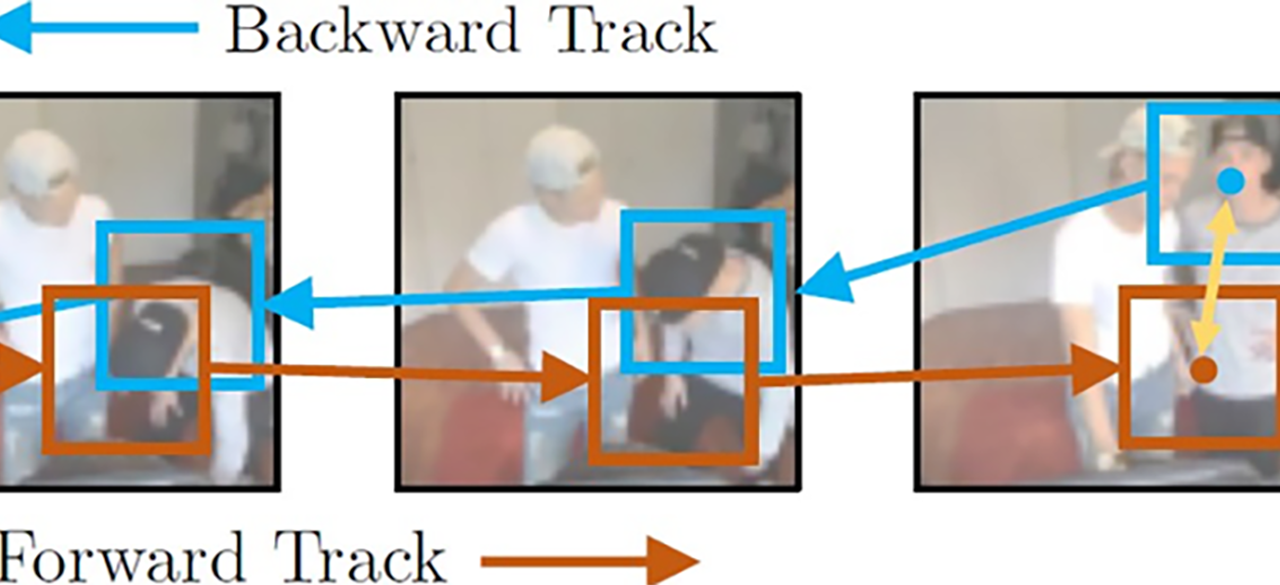
ABOUT THE PROJECT
At a glance
In this project, we propose methods for better ways of training deep models to force them to generalize to diverse real data scenarios. The idea is to make the learning algorithms work harder at training time by using self-supervision to discover the regularities in the data, instead of just memorizing the training set, as is often done now with supervised learning.
In 2021, we propose to continue harnessing the power of temporal self-supervisory signal found in unlabeled video data (such as driving videos). We plan to use the cycle-consistency loss on palindrome videos for training models that will learn to perform tracking and long-range optical flow without any supervision. Because our models do not use any supervision, we will also use them at test time, which will allow us to be more robust to smooth domain changes (e.g. time-of-day, weather, urban vs. rural, etc).
principal investigators | researchers | themes |
---|---|---|
Alexei (Alyosha) Efros | self-supervised learning, video processing, cycle-consistency, test-time training |