Model-Based Reinforcement Learning Approach for Trajectory and Intent Prediction of Vulnerable Road Users
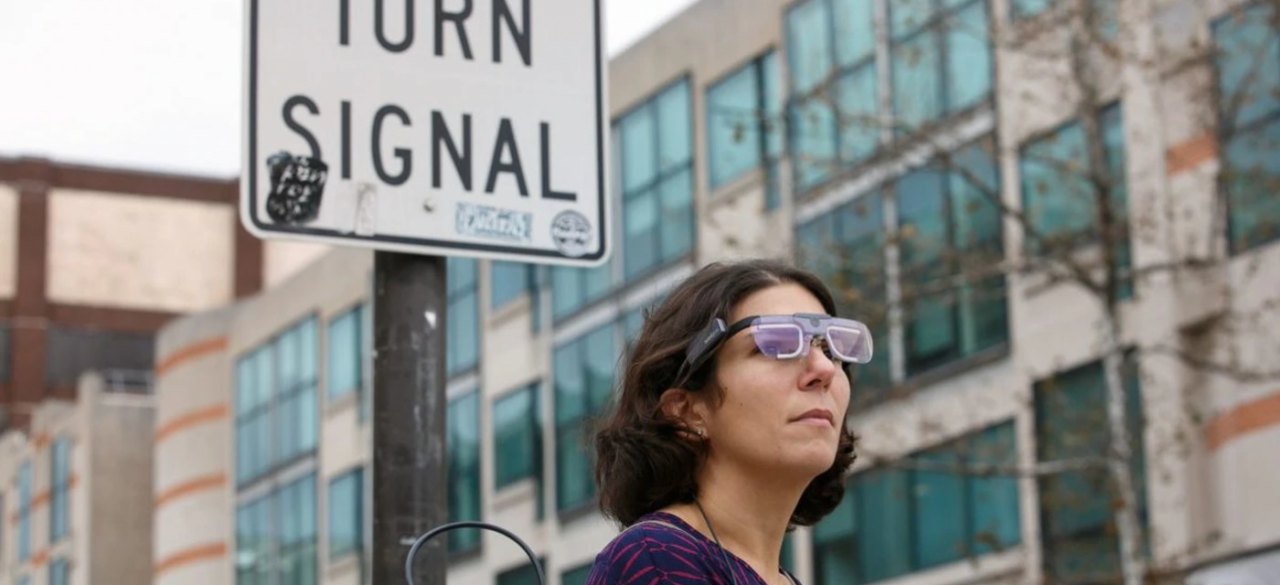
ABOUT THE PROJECT
At a glance
One of the key challenges surrounding the development and deployment of autonomous vehicle (AV) systems revolve around assurances of safety, not only for users of the AVs, but also for individuals within the vicinity of the device. This is particularly the case for vulnerable road users (VRUs) like bicyclists and pedestrians, whose safety is immediately at risk if an AV system were to misjudge their intent and future trajectory. As a result, understanding the behavior of VRUs in the context of interactions with AVs is a crucial milestone before the deployment of higher levels of automation. In fact, AVs will only be deployable if they are proven to be able to communicate and navigate complex interactions with other road users in a safe and efficient manner. As such, there is a need for stronger and better models to predict and forecast the intents of VRUs to a high degree of accuracy, particularly in situations where actions aren’t easily predictable. We propose to advance and improve such models using a Model-Based Reinforcement Learning (RL) approach to predict the intent of pedestrians and bicyclists in complex urban environments.
Many of the current techniques used to predict pedestrians’ intentions to train autonomous driving focus on only using external physical factors detected by the AVs’ sensors to generate trajectory predictions. In reality, however, when humans observe actions of others, they carry out inferences about why the others acted the way they did and what this implies about their view of the world. We plan to address this discrepancy through a collaboration with researchers at the Center for Safe Mobility (CSM) at the University of Pennsylvania, led by Professor Megan Ryerson. Through CSM, Professor Ryerson used wearable glasses to collect bicyclists’ and pedestrians’ eye-tracking data . The glasses are equipped with an outward-facing camera that captures videos of the environment as seen from the pedestrians’ and bicyclists’ perspectives, inward-facing sensors that capture pupil position, dilation, and gaze orientation, as well as a gyroscope and accelerometer to measure head movement, angle, and velocity. This data presents a great opportunity to learn about the internal state of VRUs by studying the eye-tracking information, and modeling both how the internal state of those users is affected by external factors (e.g. infrastructure, proximity to vehicles, etc.), as well as how their internal state affects their future actions.
principal investigators | researchers | themes |
---|---|---|
Joan Walker | Hassan Obeid Megan Ryerson | Model-Based Reinforcement Learning, Intent and Trajectory Prediction, Pedestrian Safety, Autonomous Vehicles, Deep Learning, Vulnerable Road Users |