Hybrid Human Driver Predictions
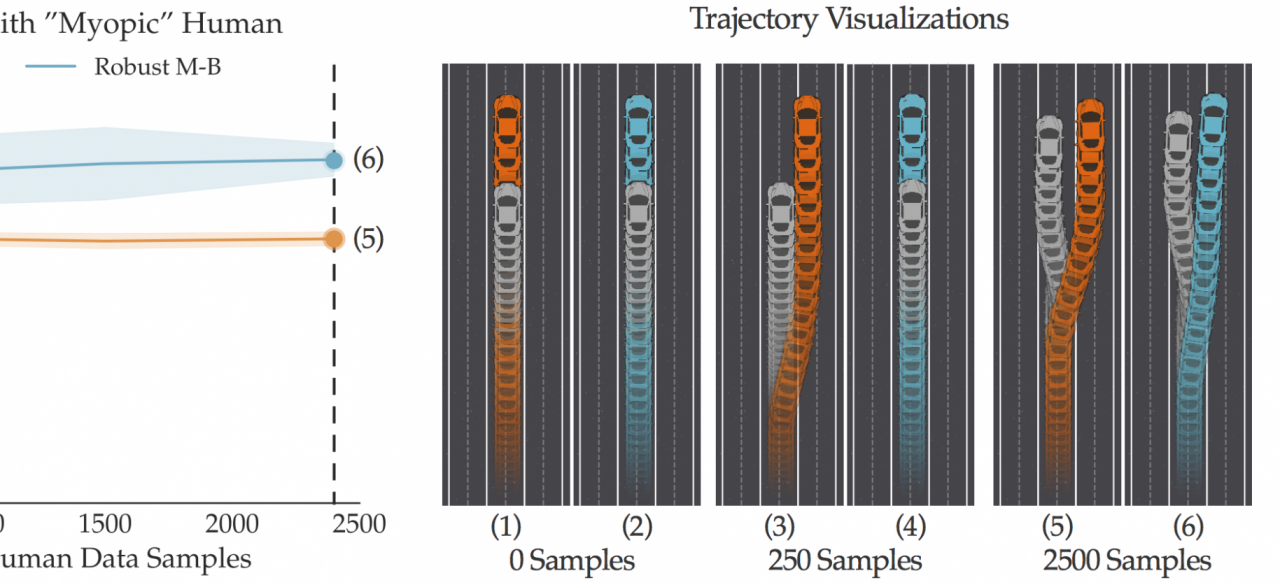
ABOUT THE PROJECT
At a glance
Our goal is to enable cars to make predictions about human behavior accurately -- predictions that adjust with the actions the autonomous car plans to take, enabling closed-loop interaction. There are two schools of thought on this: 1) end to end approaches for imitation learning that have low bias but have trouble in low sample complexity regimes; 2) "theory-of-mind" approaches that make assumptions about human behavior as intent-driven and rational, leveraging optimization and Inverse Reinforcement Learning to generate predictions; these have high bias but low variance. Neither is accurate enough, and that makes prediction one of the largest bottlenecks to scaling autonomous driving. In this project, we analyze how to combine these methods to get the best out of both worlds. We are in a unique position to tackle this incredibly important problem for autonomous driving: we have experience in both techniques, and are building on our prior work over this past year that characterizing when each technique works best.
principal investigators | researchers | themes |
---|---|---|
Anca Dragan | imitation learning, inverse reinforcement learning, model-based and model-free RL |