Efficient Model for Large-Scale Point Cloud Perception
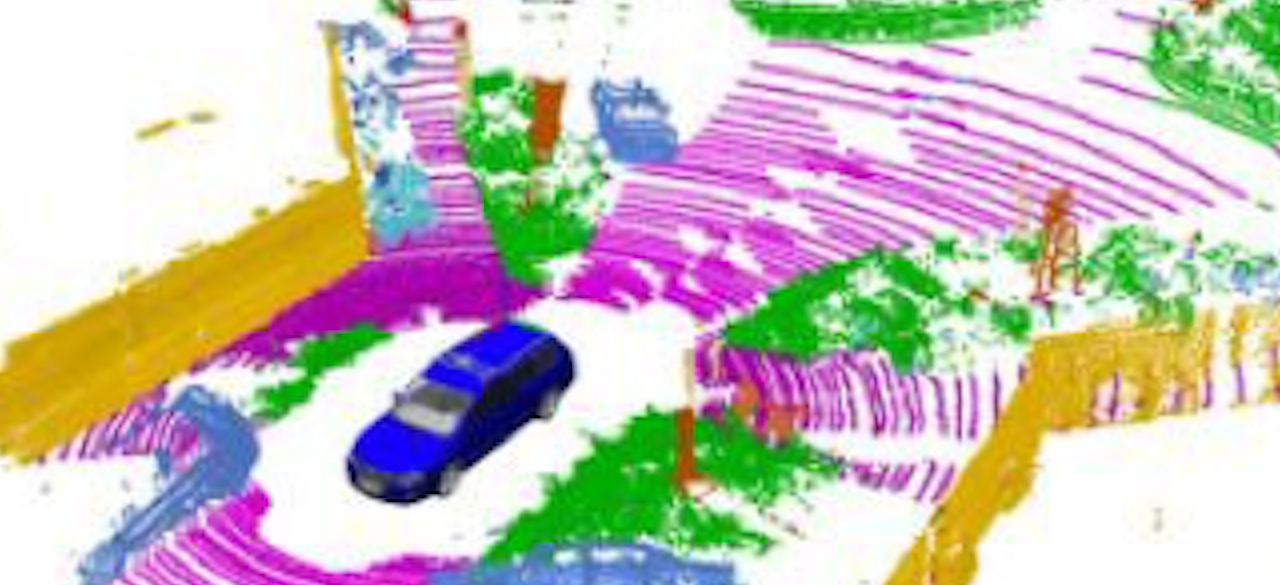
ABOUT THE PROJECT
At a glance
Semantic scene understanding is crucial for autonomous vehicles since a fine-grained understanding of their surroundings is necessary for perceptions and decisions. Previously, significant progress has been made in 2D image-based semantic segmentation and object detection. For 3D point clouds, however, accurate, robust, and fast perception algorithms are still to be explored. LiDAR point cloud plays an important role in autonomous driving since it provides rich 3D information and robust features in a challenging environment compared with cameras.
In this project, we aim to tackle efficiency problems of general point cloud processing including point-wise segmentation and object detection. Different from other proposals that focus on improving efficiency from NAS, model compression and quantization, we delve deep into the attributes of a point cloud (remission, range, coordinate) and the relations between different perception tasks, e.g., segmentation results provide point-wise labels that can be directly used for detection tasks.
PRINCIPAL INVESTIGATORS | RESEARCHERS | THEMES |
---|---|---|
Masayoshi Tomizuka Kurt Keutzer | Amir Gholami | LiDAR |