3D Object Detection with Temporal LiDAR data for Autonomous Driving
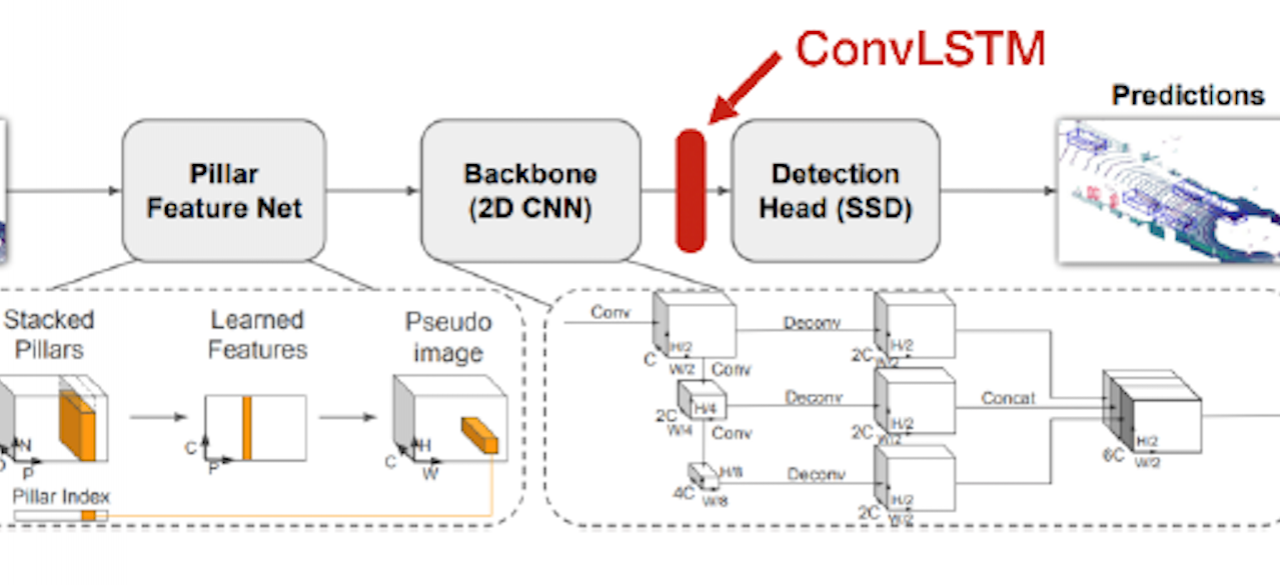
ABOUT THIS PROJECT
At a glance
A crucial task in Autonomous Vehicle applications is the ability to accurately detect and infer details about the surrounding objects accurately, and in a timely fashion. Among existing sensors used for 3D object detection, LiDAR results in highest distance accuracy, and works under low light conditions such as nighttime. Furthermore, at least for the KITTI datasets, LiDAR based systems outperform all other sensors, There have been two recent developments that have made LiDAR more attractive than ever before. One has to do a significant drop in price in newly introduced LiDAR systems, such as the Velabit by Velodyne with a price point of $100. The second has to do with the availability of labeled temporal LiDAR datasets by Waymo and NuTonomy as of mid 2019. This has opened up exciting research opportunities for development of temporal LiDAR algorithms for 3D object detection, prediction, and fusion of temporal LiDAR data with other sensors such as video. In the previous cycle, we modified an existing architecture by incorporating an LSTM module to deal with recurrent nature of temporal LiDAR. We demonstrated experimentally that the mAP for cars and pedestrians for our modified architecture is 6% and 5% higher than the original architecture. In this cycle, we explore the use of transformer networks in 3D object detection using temporal LiDAR data. We will also develop a joint self-supervised moving object segmentation and scene flow estimation for temporal LiDAR with application to autonomous driving. Scene flow and object segmentation, while seemingly separate problems, are closely intertwined. Previous works have used scene flow to segment moving objects. Furthermore, because most motion in a scene is rigid, the scene flow can also be parameterized as a rigid body transformation for each detected object. In this work, we intend to use these two ideas to perform joint, self-supervised moving object segmentation and scene flow estimation by developing novel loss functions that enforce consistency between the scene flow and object segmentation predictions. We speculate that jointly training the two networks allows each of them to perform better on their respective tasks. We use KTTI dataset to demonstrate the performance of our results. We plan to leverage over $200,000 cloud computing credit with AWS, Azure to run our training algorithms.
principal investigators | researchers | themes |
---|---|---|
Avideh Zakhor | Temporal LiDAR, 3D Object Detection, Self-supervised object Segmentation, autonomous driving, Transformer Network |