Automatic Inference of Lane Topology and Connecting Geometry for Scene Understanding, High-Definition Map Construction and Representation
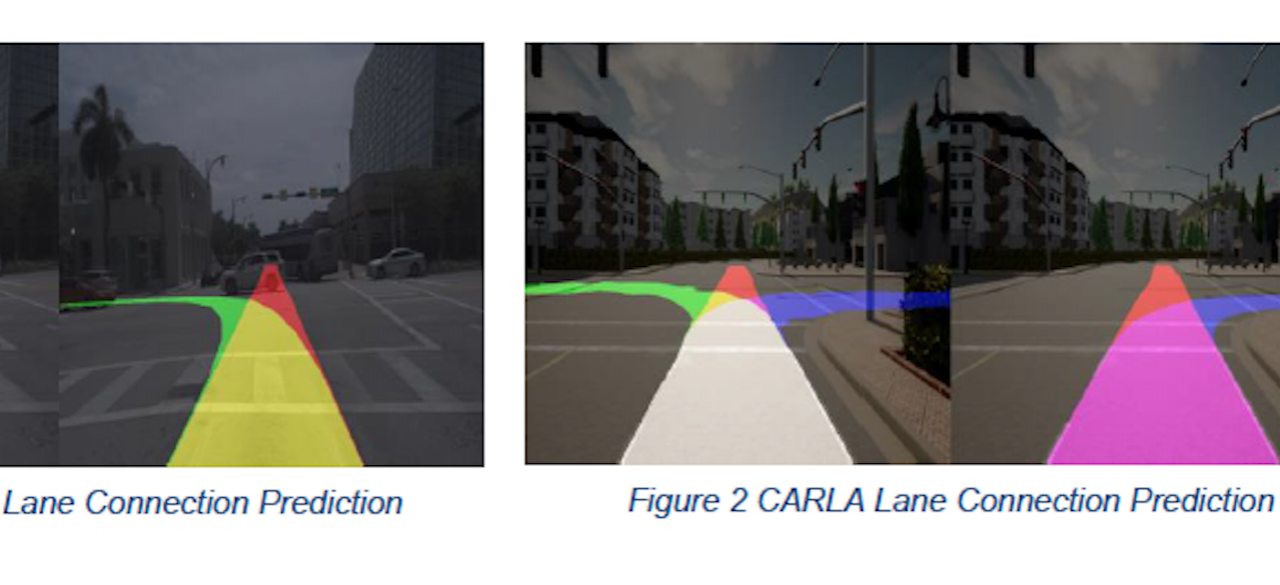
ABOUT THIS PROJECT
At a glance
Autonomous vehicles need to understand the driving scenes with semantics such as drivable areas, lanes and intersections. For vehicles to navigate in a complicated urban environment, it is also important to understand the lane relationships. More specifically, it is critical for an autonomous vehicle to know lane connection topologies and geometries on the roads or in the intersections. The aforementioned road semantics and lane topology can be further incorporated into a high-definition (HD) map. However, constructing such HD maps with this information in large scale by humans can be extremely overwhelming. Moreover, autonomous vehicles need to extract the road structure online when there is no HD map provided. Therefore, automatic lane topology inference is crucial to enable scalable full autonomy.
Furthermore, the preferred reference paths of human drivers may not strictly follow the “ideal” lane centers. For example, different drivers may turn to different lanes at an intersection. Therefore, we need a novel representation of the implicit lane topology/geometry either offline (for the HD maps) or online (for scene understanding). In this project, we aim at automatically extracting lane relationships from onboard sensors such as LiDARs and cameras, and propose novel representation of the implicit connection rules obtained via human prior and motion data.
principal investigators | researchers | themes |
---|---|---|
Masayoshi Tomizuka | Wei Zhan | HD map, scene understanding, map construction and inference |
This project is a continuation of: "Automatic Semantics Extraction and Representation for High-Definition Map Construction and Scene Understanding".