Analysis and Synthesis of Road Vehicle Conflicts using Vehicle Trajectory Data
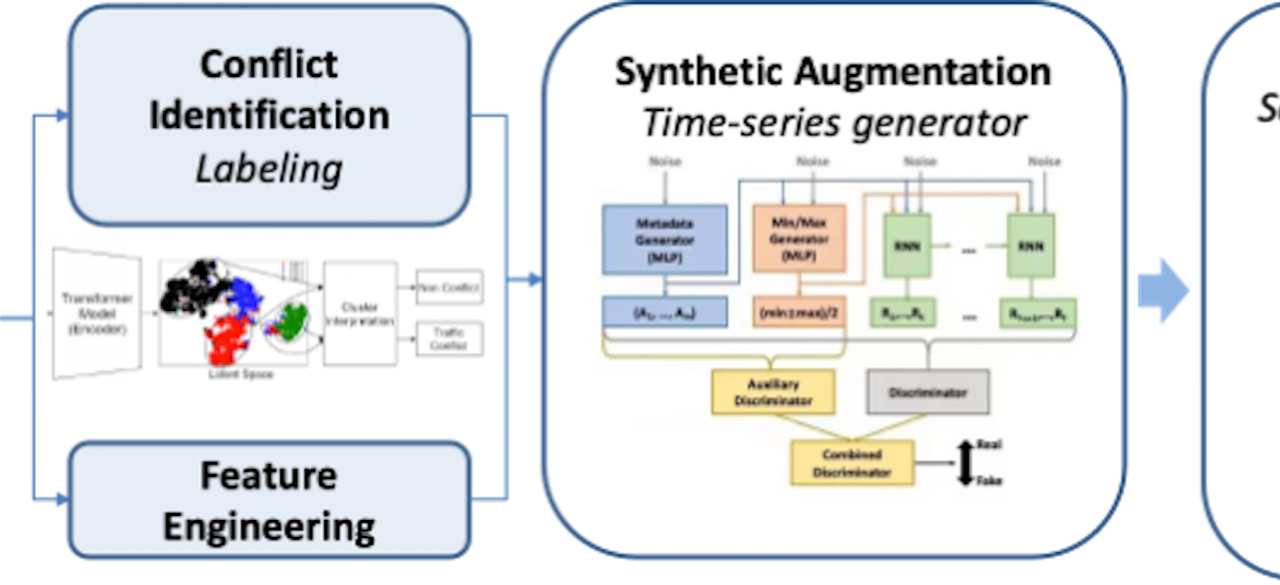
ABOUT THE PROJECT
At a glance
Progress in traffic safety, in both the current system and the increasingly automated future, depends on shifting toward a more proactive approach in which hazardous conditions are foreseen and mitigated prior to the occurrence of crashes. In the context of vehicle-to-vehicle collisions, the precursor condition is often referred to as a “conflict.” It is therefore of interest to analyze the incidence of vehicle conflicts. Given that conflicts are relatively rare, a research challenge is to maximally exploit the limited trajectory data available to analyze and predict conflict occurrence. We propose to develop the data-driven real-time risk predictive framework, using real-world driving data and advanced machine learning techniques, to continuously monitor and identify potential risks of vehicle-to-vehicle conflicts. This framework incorporates unsupervised learning for conflict identification, deep generative models to augment the conflict samples, sequential learners to make predictions, and data streaming pipeline for real-time deployment. This basic research can mature into capabilities with a variety of use cases for both conventional and automated vehicles.
principal investigators | researchers | themes |
---|---|---|
safety evaluation, risk indicators, systemic risks, traffic conflict, synthetic augmentation |