Cross-Domain Self-Supervised Learning for Adaptation and Generalization
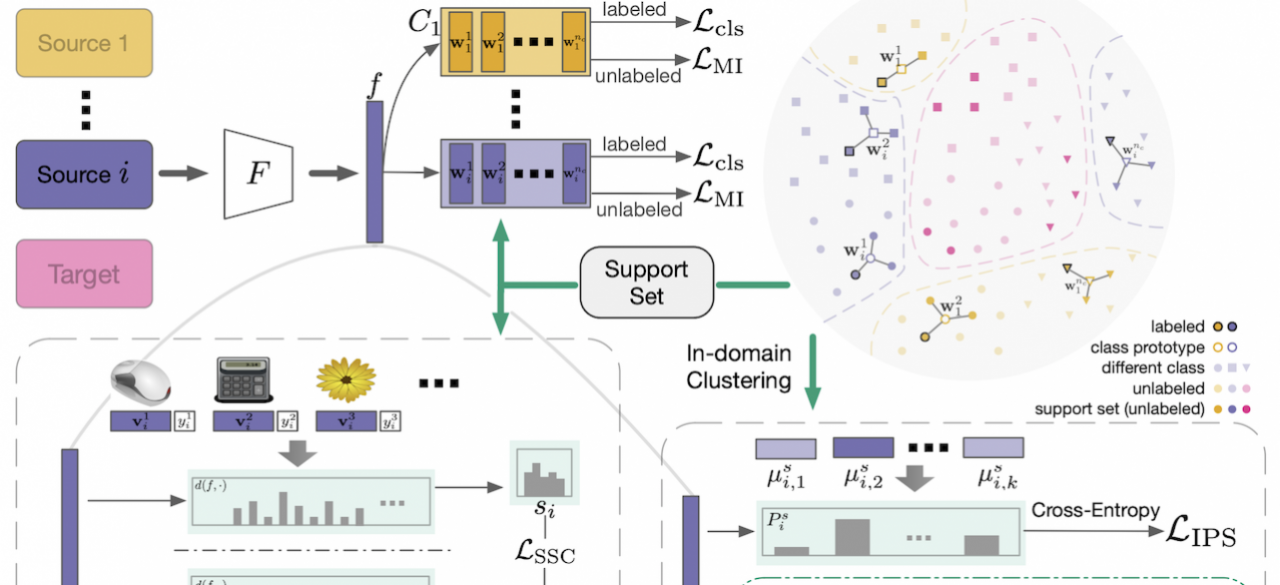
ABOUT THE PROJECT
At a glance
Most machine learning algorithms have achieved remarkable performance in various autonomous driving applications. Despite high accuracy, deep neural networks trained on specific datasets often fail to generalize to new domains owing to the domain shift problem. In our previous works, we proposed cross-domain self-supervised learning methods and demonstrated their effectiveness on few-shot unsupervised domain adaptation and multi-source few-shot domain adaptation. With cross-domain SSL, the performance boost on domain adaptation is significant. In this project, we aim to develop new cross-domain SSL methods for more complex perception tasks, e.g. object detection and semantic segmentation. We plan to perform cross-domain SSL by finding the region-level relationships between dense features. In addition, we plan to further develop better methods by finding the causal structures and low-dimensional structures under the data generation process. After performing cross-domain self-supervised learning for object detection and semantic segmentation, we propose to leverage the learned representations and train models to achieve better domain adaptation / generalization properties.
principal investigators | researchers | themes |
---|---|---|
Chenfeng Xu | Self-supervised Learning, Domain Adaptation, Domain Generalization |