3D Object Detection Enhanced by Temporal Multi-View Input
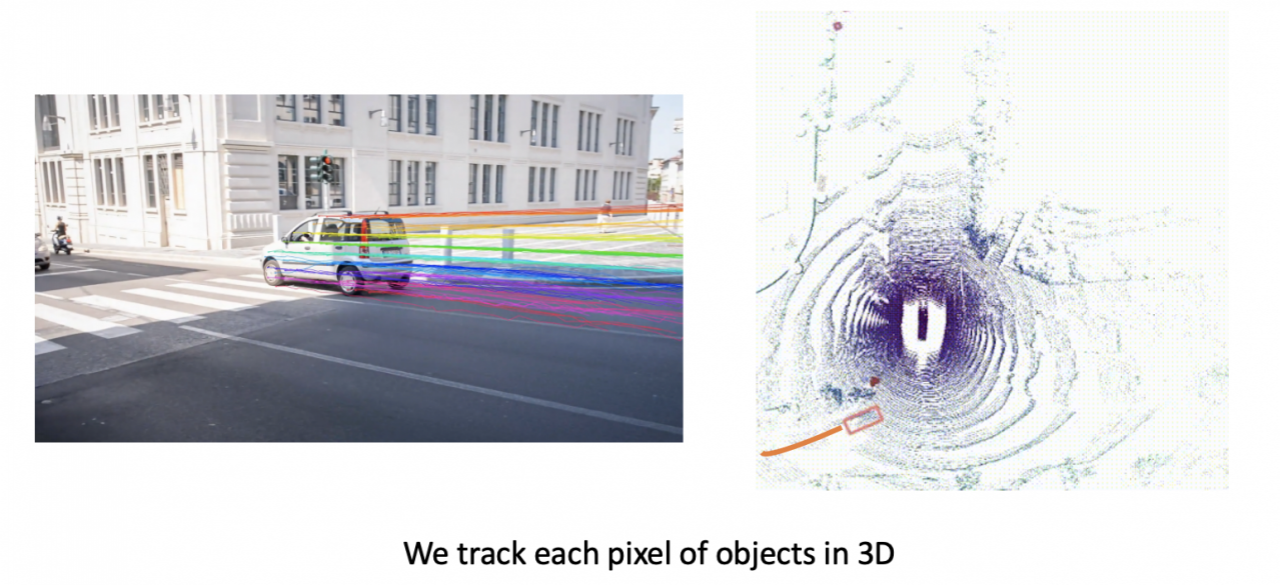
ABOUT THIS PROJECT
At a glance
3D Object detection plays a key role in autonomous driving. However, current methods insufficiently integrate the temporal information into the 3D object detection, resulting in the unsatisfactory performance. Our previous work, “Time will tell,” [1] reformulates the 3D object-detection based on images into temporal multi-view stereo, which achieves the state-of-the-art on the problem of visual 3D perception on the nuScenes dataset. This proposal is an extension of [1], aiming at further improving the 3D object detection and enabling 3D auto-labeling. We plan to improve the current work in three ways:
1) In [1], although the reformulation of temporal multi-view stereo significantly improves the performance, we observe that detecting fast-moving objects is still challenging. As the sensor calibration is noisy, the performance would degenerate more drastically.
2) [1] is the solution of pure image 3D object detection, while autonomous vehicles are equipped with other sensors like LiDAR and Radar. The different sensors have their own advantages, which can be utilized for enhancing the accuracy, the robustness, and the generalizability of the 3D object detection and our work seeks to integrate these sensors.
3) For offboard 3D object detection [2] which can be used for 3D auto-labeling, incorporating both past frames and future frames could lead to an extremely long-time information fusion. This could largely improve the performance of 3d detection.
To summarize, we propose a flexible temporal multi-sensor fusion solution to 1) improve fast moving object detection under noisy sensor calibration, 2) make full use of existing sensors to enable accurate, robust, and general 3D detection to enable more capabilities such as very close obstacle detection and narrow scene perception etc., and 3) deploy our model into the auto-labeling pipeline. While not all autonomous driving cars are equipped with LiDAR and Radar, our solution would be trained on all the available sensors such that the sensor knowledge can be distilled into the model, during inference, our model is flexible enough to accept any sensor input.
Principal investigators | researchers | themes |
---|---|---|
Chenfeng Xu | 3D detection, multi-view, sensor fusion, auto-labeling |