Advisable Deep Driving
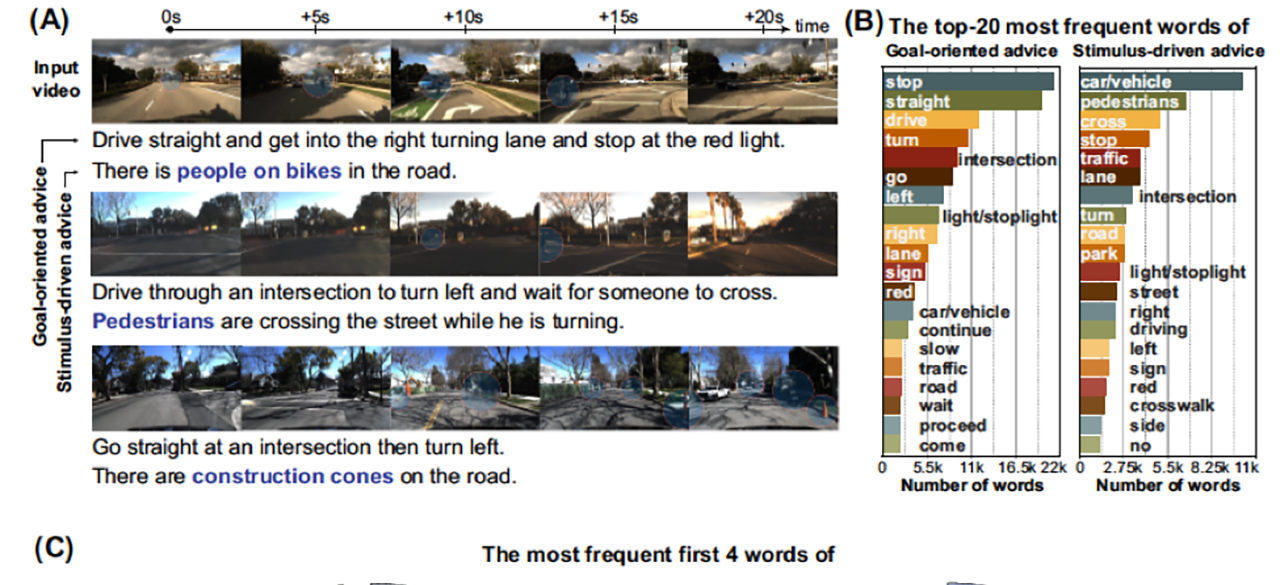
ABOUT THE PROJECT
At a glance
This project builds on our earlier work on explainable vision-based deep driving models from ICCV 2017, NIPS 2017 and ECCV 2018. There we showed that attention-based deep driving models can provide either visual or textual explanations of their behavior in terms of objects in the scene. Explanations can help build user trust and acceptance for self-driving vehicles and help users anticipate the system’s behavior in new situations. In the course of this work, we identified some limitations of the data-driven models that we trained using deep networks. Namely that the models are semantically-shallow: while they are capable of recognizing objects such as stop lights or pedestrians, they have limited understanding of these objects beyond their role in predicting driver behavior. Deep models typically under-attend to pedestrians and have no awareness of risky situations, such as pets or children’s toys in the street. Finally, models trained using only human imitation data will reproduce certain driver bad habits, such as failing to stop fully at stop signs. For this reason, we are developing advisable deep models. Such models are capable of acting with full autonomy, but can also modify their behavior in response to textual user input. We prefer the term “advice” to “commands” since the deep model still bears ultimate responsibility for driving safety, and may or may not act according to the advice.
principal investigators | researchers | themes |
---|---|---|
John Canny | deep driving models, autonomous vehicles, human computer interaction, explainable AI, advisable models |