Augmenting Autonomous Vehicle Technology with I2V Information
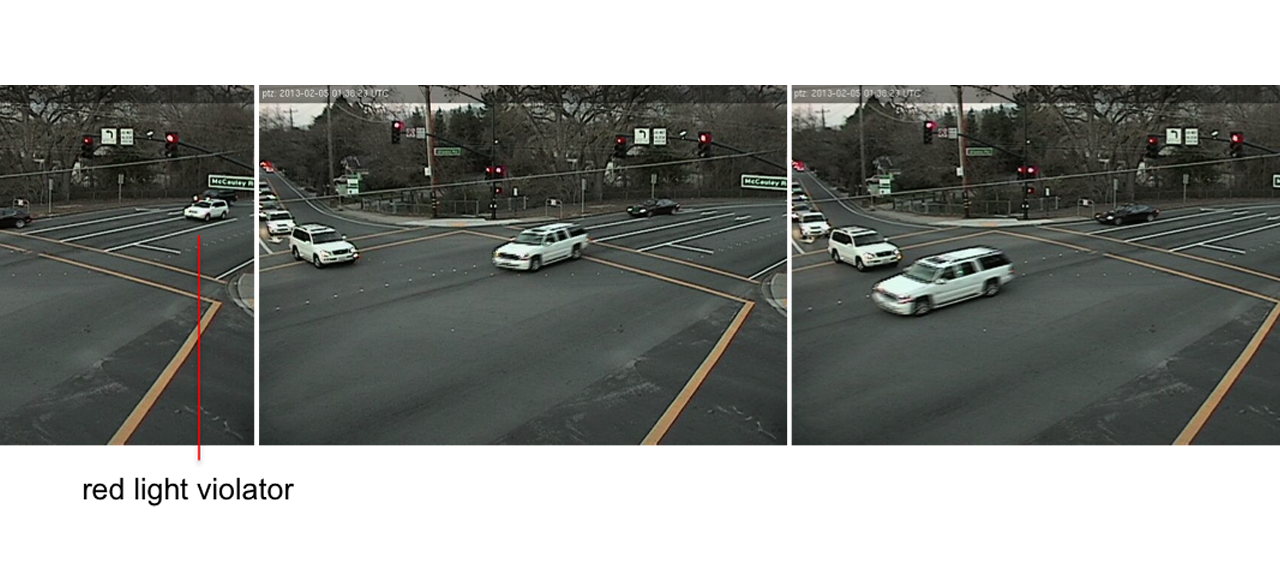
About this Project
At a glance
According to the National Highway Traffic Safety Administration (NHTSA), red‐light running is the leading cause of urban crashes: an average of 3.2 red light violations occur per hour causing over 1.8 million accidents at intersections, resulting in 118,000 injuries and over 700 fatalities per year. Almost all drivers (96%) fear being struck by a red-light runner, while the majority of Americans (56%) admit to running red lights. This prompts the need for a mechanism of early detection of potential violators and a warning signal in vehicles equipped with driver assistance systems. Since deployment of connected and autonomous cars will happen gradually, there will be a period of time during which driverless vehicles will be sharing the road with human drivers, some of whom are inattentive or impatient. Therefore, the ability to predict intersection maneuvers of potential violators is an important safety requirement for autonomous vehicles.
The high level workflow of the semi-autonomous vehicle decision support system has three steps. Step 1 comprises video processing and estimating vehicle trajectories from video frames. Step 2 involves extrapolating the retrieved vehicle trajectories to the near‐term future and flagging projected violators. Control decisions based on the information from step 2 are made in step 3. This project focuses on step 2 of the described workflow: development of the deep learning algorithm and related software for prediction and classification of vehicle trajectories at intersections. The team will train this algorithm using high-resolution traffic measurements collected from an instrumented intersection in Danville, CA.
The high level workflow of the semi-autonomous vehicle decision support system has three steps. Step 1 comprises video processing and estimating vehicle trajectories from video frames. Step 2 involves extrapolating the retrieved vehicle trajectories to the near‐term future and flagging projected violators. Control decisions based on the information from step 2 are made in step 3. This project focuses on step 2 of the described workflow: development of the deep learning algorithm and related software for prediction and classification of vehicle trajectories at intersections. The team will train this algorithm using high-resolution traffic measurements collected from an instrumented intersection in Danville, CA.
Principal Investigators | researchers | themes |
---|---|---|
Vehicle Safety |