Auto-tuned Sim-to-Real Transfer
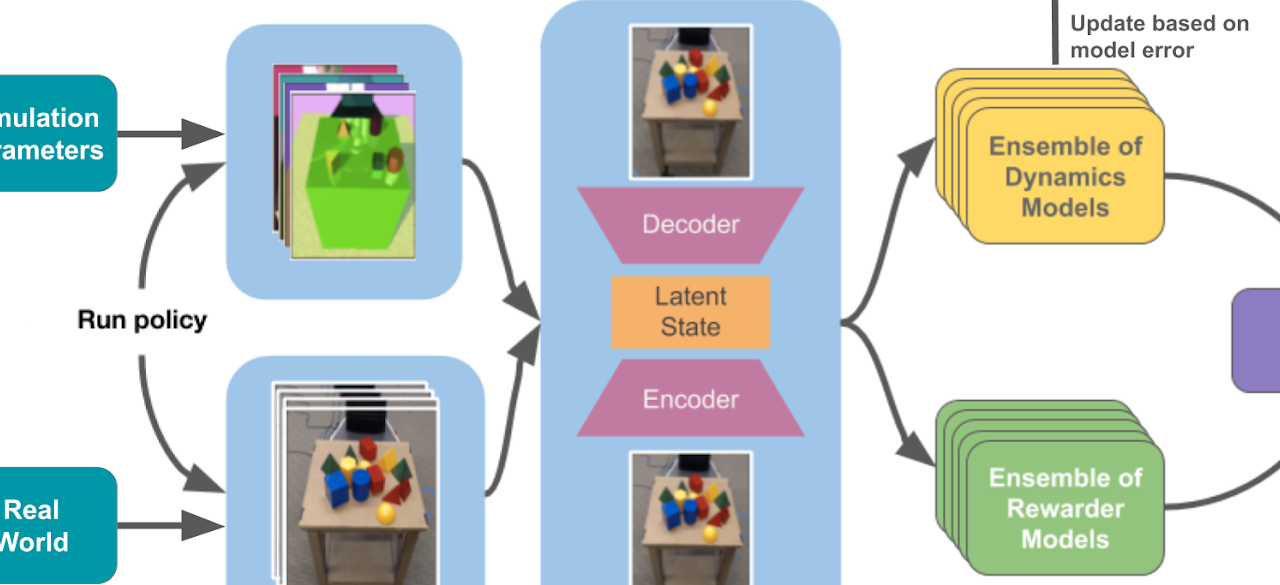
ABOUT THE PROJECT
At a Glance
Recent developments in deep learning for control has done wonders but suffers from requiring large amounts of data, which is often infeasible in the real world. Simulations tackle the data problem in many real-world applications, ranging from self-driving [1], to locomotion [2] to manipulation [3]. However, due to the ‘reality gap’ [4], which arises from the difficulties in accurately simulating properties of the real world, adaptation from simulations is challenging. One way to circumvent the reality gap is to learn directly from real data [5], but for an application such as driving, this can often be time-consuming, unsafe, and challenging when a reward function for the real-world task is unknown. Current techniques for addressing the reality gap such as system identification, domain adaptation [6], or domain randomization [7, 8] often require task-specific engineering to create simulation environments that facilitate successful transfer to the real world. However, the biggest challenge in all these techniques is the sheer amount of engineering effort to make these sim2real transfers succeed. In this proposal, our goal is to lay foundations for automating the sim-to-real effort in a way that alleviates the tedious hand-engineering needed in current approaches. We hope such an effort would accelerate the adaptation of simulated data in several real-world tasks, from self-driving cars to robots in homes.
We propose a task-agnostic model-based reinforcement learning (RL) method that will close the reality gap by combining unlabelled real-world data with domain-randomized simulated data during training
PRINCIPAL INVESTIGATORS | RESEARCHERS | themes |
---|---|---|
Pieter Abbeel | Advanced machine learning for intelligent autonomy, Advanced robotics and manipulation, Functionality and applications for automated driving |