Exploiting Environment and In-Vehicle Information from Multi-Sensors for Autonomous Driving Policy Adaption
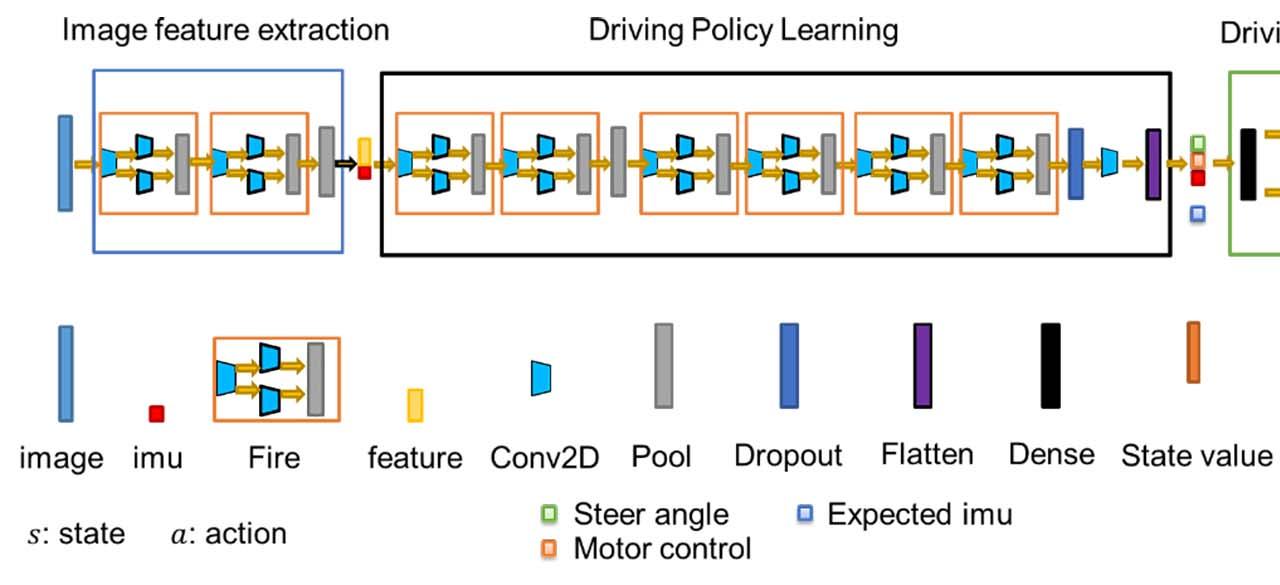
ABOUT THE PROJECT
At a glance
Many self-driving models have been investigated based on the concept of visuomotor end-to-end solutions, which take sequences of images as inputs to infer the steering and throttle or braking controls. In the visuomotor-based solution, the model implicitly learns the driving environment from the input images then executes corresponding lateral and longitudinal controls. While the end-to-end model demonstrates a powerful application of deep learning, the model often needs to be re-trained if it is to be deployed on a new vehicle model.
To cope with this issue, we propose that the information from environment sensors, such as cameras and lidar, and in-vehicle sensors, such as inertial measurement units, are to be fused in the driving policy learning process. Both visual learning for trajectory planning and sensor fusion for control feedback are taken into account in the calculation process of driving policy adaptation. One possible methodology to adopt the proposed idea is reinforcement learning, in which, state-action pairs are used to describe the system behavior and each state-action pair is associated with a reward to represent the significance to the system. The information from the environmental sensors and in-vehicle sensors are fused through reward functions to relate to safety and efficiency.
We present a deep reinforcement learning framework for the proposed driving policy adaption. The network includes three sub-network modules: the image feature extraction sub-network, the driving policy learning sub-network, and the driving policy sub-network. To deal with the continuous state and action spaces, the wire-fitting interpolation method is adopted to approximate the local optimal of the long-term reward function.
Principal investigators | Researchers | themes |
---|---|---|
Ching-Yao Chan | Yi-Ta Chuang | Sensor Fusion Driving Policy Adaptation |