Few-Shot Imitation and Embodiment Transfer
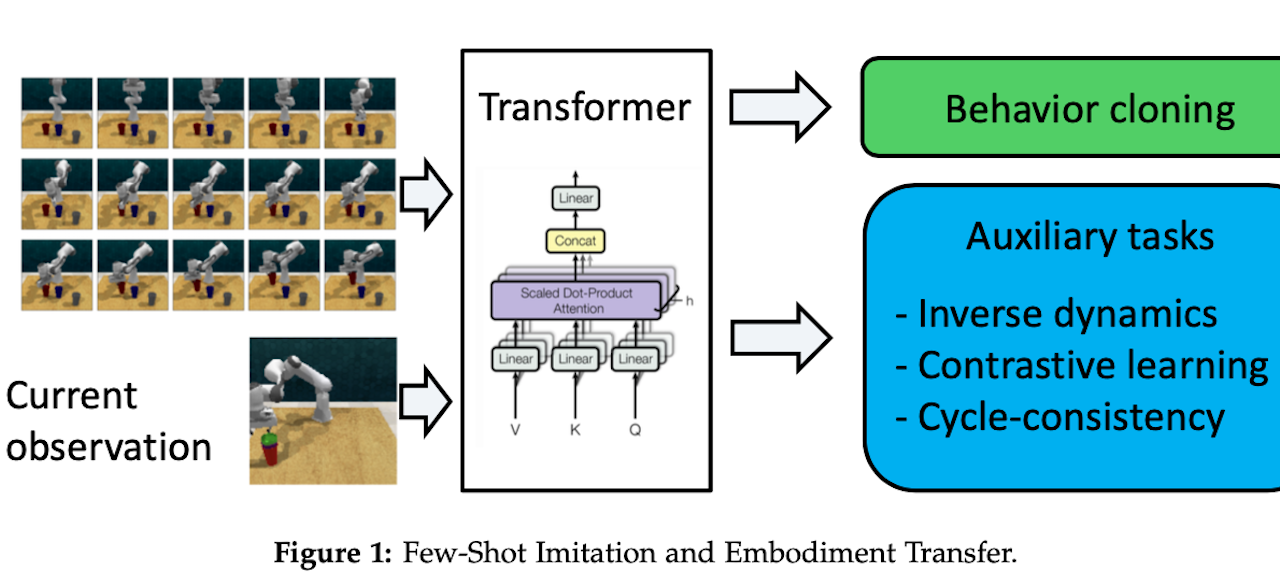
ABOUT THE PROJECT
At a glance
Imitation learning has been successfully allowed agents to perform control tasks from raw, high-dimensional input such as camera imagery [1–3]. However, if we take a closer look at these breakthroughs, there are some fundamental limitations: Past work has required many demonstrations on tasks very similar to the final downstream task. Real-world tasks are much more unstructured and it might be hard to collect many demonstrations. Additionally, the demonstration may come from an agent with different morphology and physical appearance (e.g. different robot or human). In this proposal, our goal is to scale imitation learning to solve unstruc- tured real-world challenges with a few demonstrations. We hope such an effort would accelerate the adaptation of imitation learning in several real-world tasks, from locomotion controls to robot manipulation.
In this research, for few-shot imitation and embodiment transfer, we will investigate advanced attention-based neural architectures, which could allow the agent to generalize to conditions and tasks unseen in the training data. Also, we will leverage unsupervised representation learning like contrastive learning and cycle-consistency to extract the few-shot signal and invariant features from demonstrations with domain gaps. We hypothesize that this new framework will enable agents to solve unstructured real-world challenges using a few demonstrations via imitation learning.
principal investigators | researchers | themes |
---|---|---|
Pieter Abbeel | Imitation Learning, Self-Attention, Few-shot Learning, Robotic Manipulation |