Generic Motion Generation and Comprehension with Social Interactions
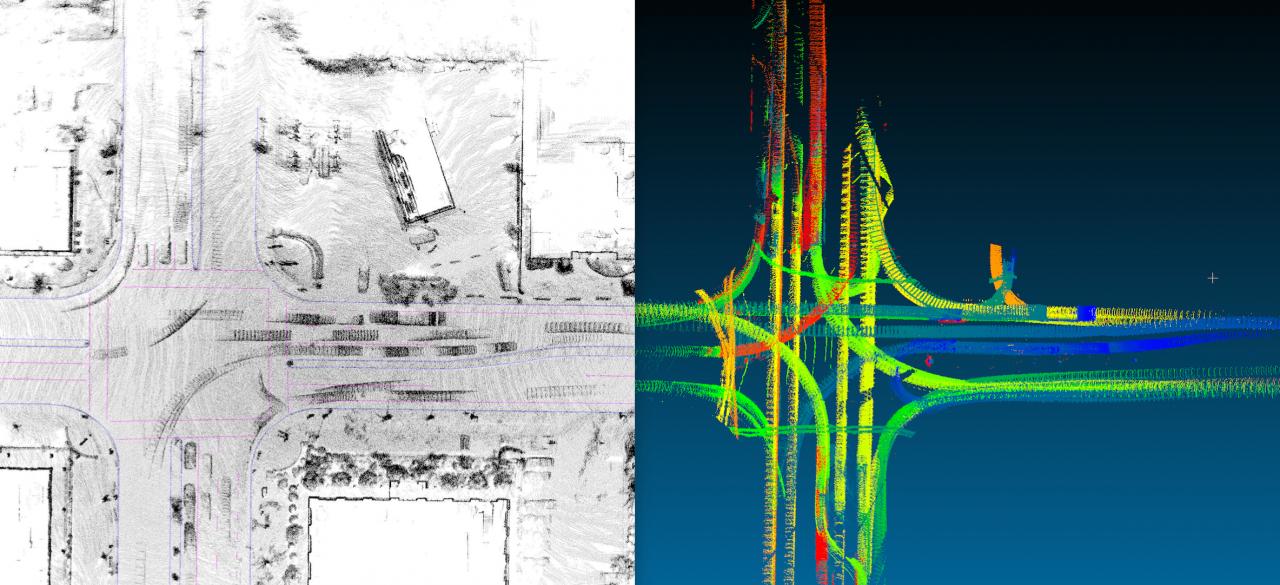
With point cloud data obtained, we build the high-definition map of an intersection and extract the motions of the vehicles and pedestrians at this intersection with the help from Ehsan Javanmardi and Mahdi Javanmard.
ABOUT THE PROJECT
At a glance
Learning-based methods can improve the comprehension of the behavior of others by observing motions and interactions in real-world driving scenarios, but it is hard to incorporate domain knowledge such as accurate models of vehicle motions and to guarantee collision avoidance. By contrast, model-based methods, such as planning based on optimization with hard constraints or planning-based prediction, are relatively easy to incorporate domain knowledge and capable of generating collision-free and feasible motions, but lack flexibility to improve the comprehension via observation.
Recently, researchers attempted to integrate learning-based and model-based methods to achieve accurate comprehension under social interaction and safe motion generation for autonomous driving, such as a recent paper from Mobileye combining policy net and constrained optimization. However, the input features and output indicators of the learning-based method, which describe the state of the environment and desires of others, respectively, are dedicated for a specific scenario and cannot be generalized to others. Moreover, the learning framework can hardly improve the comprehension incrementally with small amount of new observations. Also, the domain knowledge in the model-based planner cannot facilitate the comprehension from the learning-based model.
In this project, we will develop general input features and output indicators for learning-based model which enable generic comprehension of the driving policy in various kinds of scenarios. Model-based methods such as planning-based prediction and intention recognition developed in our first-year work will be used as a baseline to facilitate the learning-based comprehension method. With fairly good planning-based predictions, we will design learning-based model which can improve the comprehension incrementally with small amount of observations. With the human-like prediction via integrating learning-based and model-based methods, we will also improve the non-conservatively defensive strategy to balance between the driving quality and expectation of other human drivers to achieve safe, efficient and human-like motion generation under social interactions. Moreover, we will extend the Constrained Policy Net proposed to learn stochastic driving policy of human drivers from real world motion data in a variety of scenarios, while constraints for vehicle feasibility and collision avoidance are still satisfied by integrating the domain knowledge from a model-based expert planner.
Recently, researchers attempted to integrate learning-based and model-based methods to achieve accurate comprehension under social interaction and safe motion generation for autonomous driving, such as a recent paper from Mobileye combining policy net and constrained optimization. However, the input features and output indicators of the learning-based method, which describe the state of the environment and desires of others, respectively, are dedicated for a specific scenario and cannot be generalized to others. Moreover, the learning framework can hardly improve the comprehension incrementally with small amount of new observations. Also, the domain knowledge in the model-based planner cannot facilitate the comprehension from the learning-based model.
In this project, we will develop general input features and output indicators for learning-based model which enable generic comprehension of the driving policy in various kinds of scenarios. Model-based methods such as planning-based prediction and intention recognition developed in our first-year work will be used as a baseline to facilitate the learning-based comprehension method. With fairly good planning-based predictions, we will design learning-based model which can improve the comprehension incrementally with small amount of observations. With the human-like prediction via integrating learning-based and model-based methods, we will also improve the non-conservatively defensive strategy to balance between the driving quality and expectation of other human drivers to achieve safe, efficient and human-like motion generation under social interactions. Moreover, we will extend the Constrained Policy Net proposed to learn stochastic driving policy of human drivers from real world motion data in a variety of scenarios, while constraints for vehicle feasibility and collision avoidance are still satisfied by integrating the domain knowledge from a model-based expert planner.
pRINCIPAL INVESTIGATORS | RESEARCHERS | THEMES |
---|---|---|
Wei Zhan, Jiachen Li, Yeping Hu | Driving Policy |