Hyperbolic Embedding for Natural Visual Scene Recognition
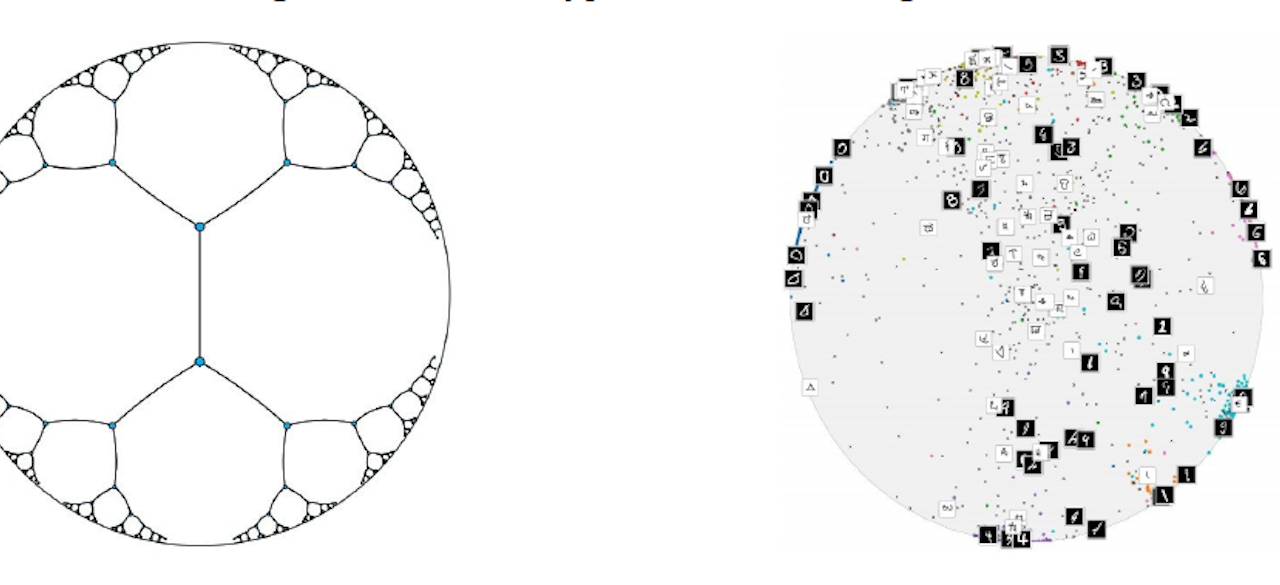
ABOUT THIS PROJECT
At a glance
Visual scene recognition is fundamentally hierarchical, whether in terms of low-level visual structures or high-level semantic concepts. Most deep learning methods develop a visual representation in the Euclidean space, which falls short of capturing such hierarchical structures, since a tree cannot be isometrically embedded into the Euclidean space. The hyperbolic space is a continuous analog of a tree structure, and it has been used in natural language processing to encode the semantic hierarchy of WordNet. However, it is unclear how to extract a visual hierarchy in a data-driven fashion, how to exploit the hyperbolic embedding for various visual tasks, and whether it would matter much at all in practice. We propose to leverage our expertise on representation learning for image classification, open long-tailed recognition, and lifelong learning, to answer these questions. Our preliminary results show an easy and significant performance gain in natural visual scene recognition with the hyperbolic embedding.
principal investigators | researchers | themes |
---|---|---|
Stella Yu | Yunhui Guo Haoran Guo Jyh-Jing Hwang Tsung-Wei Ke Xudong Wang Yubei Chen | hyperbolic embedding, Poincaré ball, long-tailed recognition, out-of-distribution detection, open-set recognition, life-long learning, continual learning, unsupervised representation learning |