Learning Dynamic Point Set Neighbourhoods for 3D Object Detection
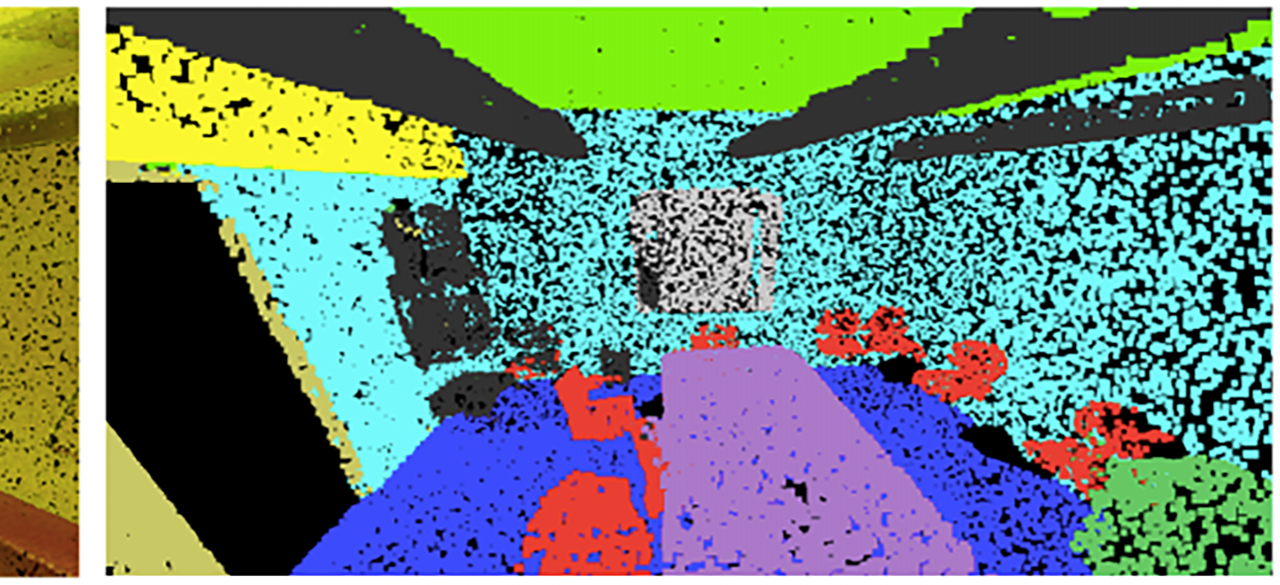
ABOUT THE PROJECT
At a glance
A self-driving car could use 3D point clouds along with 2D images to detect objects of interest such as pedestrians and vehicles faster and more accurately. In existing deep learning approaches for 3D point cloud processing, as the network gets deeper, 3D feature learning still relies on the fixed original point set neighbourhoods, unlike 2D image feature learning. We propose to achieve accurate, efficient, and robust 3D point cloud object detection by learning dynamic point set neighbourhoods at individual depth layers, by learning feature and region proposals simultaneously, and by extending such a 3D learning framework to unsupervised and semi-supervised settings.
principal investigators | researchers | themes |
---|---|---|
Stella Yu | Peter Wang Rudrasis Chakraborty | Dynamic Point Set Neighbourhood, Feature Learning, 3D Object Detection, Unsupervised Learning, Semi-supervised Learning |