Life-Long Learning to Drive by Semi-Supervised Reinforcement Learning (SSRL)
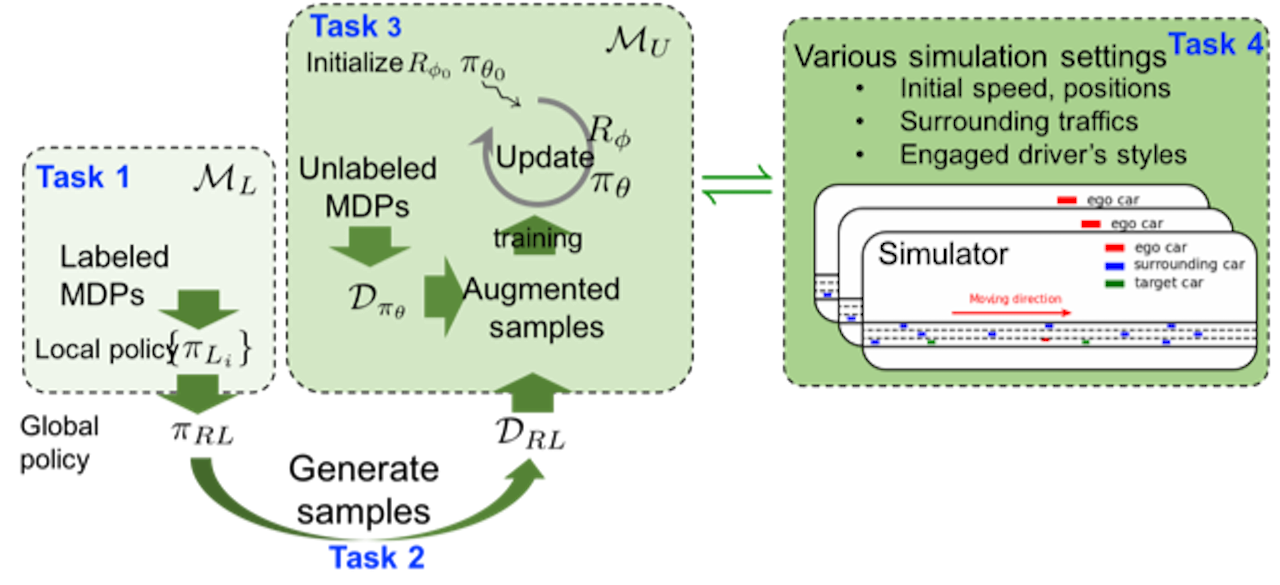
ABOUT THE PROJECT
At a glance
This is a proposed continuation of a 2019 project, which was partially funded. This proposal provides updates of the progress up to date and the research plan for the extension phase.
In this project, we aim to utilize both labeled and unlabeled datasets to enable the life-long learning ability of decision-making task for autonomous agents, by integrating the merits of semi-supervised (SS) learning and reinforcement learning (RL). In other words, the agent is trained with reward available yet insufficient data and can continue its learning from reward unavailable cases. The concept is based on an earlier work [Finn et al, 2017], where the problem is formulated as a semi-supervised reinforcement learning (SSRL) process and verified with OpenAI Gym environments. In our study, we extend it to the more challenging and practical application case of autonomous driving, i.e. decision-making task for lane changes.
principal investigators | researchers | themes |
---|---|---|
Pin Wang Ching-Yao Chan Pieter Abbeel | Semi-Supervised Reinforcement Learning, Life-Long Learning, Autonomous driving behavior |