Localization and 3D Detection via Fusing Sparse Point Cloud and Information from Visual-Inertial Navigation System
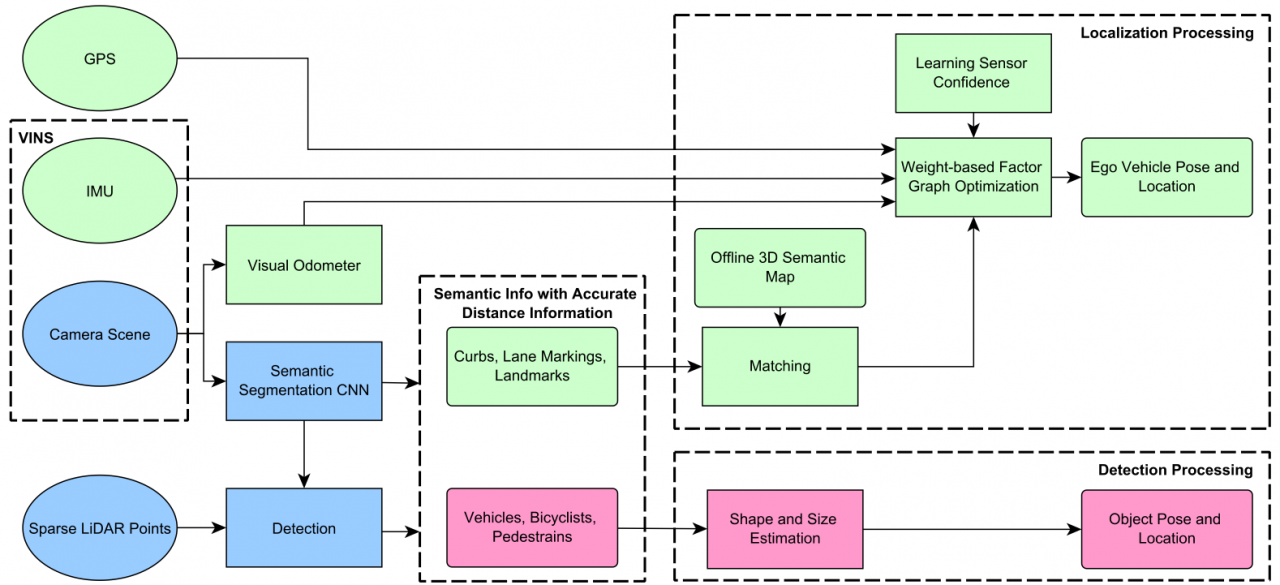
ABOUT THE PROJECT
At a glance
High-end LiDARs (with 32 laser beams or above) have been widely used in prototypes of autonomous vehicles. However, the number of beams of automotive-grade or low-cost LiDARs is still within 4-16, which produce relatively sparse point cloud. The sparsity makes it extremely challenging to achieve precise and robust localization and 3D detection. Few research efforts have been made to fuse sparse point cloud with information from cameras, GPS and IMU for localization and 3D object detection. In this project, we propose to construct a framework to precisely localize autonomous vehicles in various kinds of scenarios with factor-graph-based methods fusing sparse point cloud and information from visual-inertial navigation system (VINS) with a 3D semantic high-definition (HD) map. Moreover, a parallel-fusion pipeline will be designed specifically aiming at 3D detection problems when fusing sparse point cloud and images.
principal investigators | researchers | themes |
---|---|---|
Masayoshi Tomizuka | Wei Zhan | localization, 3D detection, sensor fusion |