Multi-Modal Self-Supervised Pre-training for Label-Efficient 3D Perception
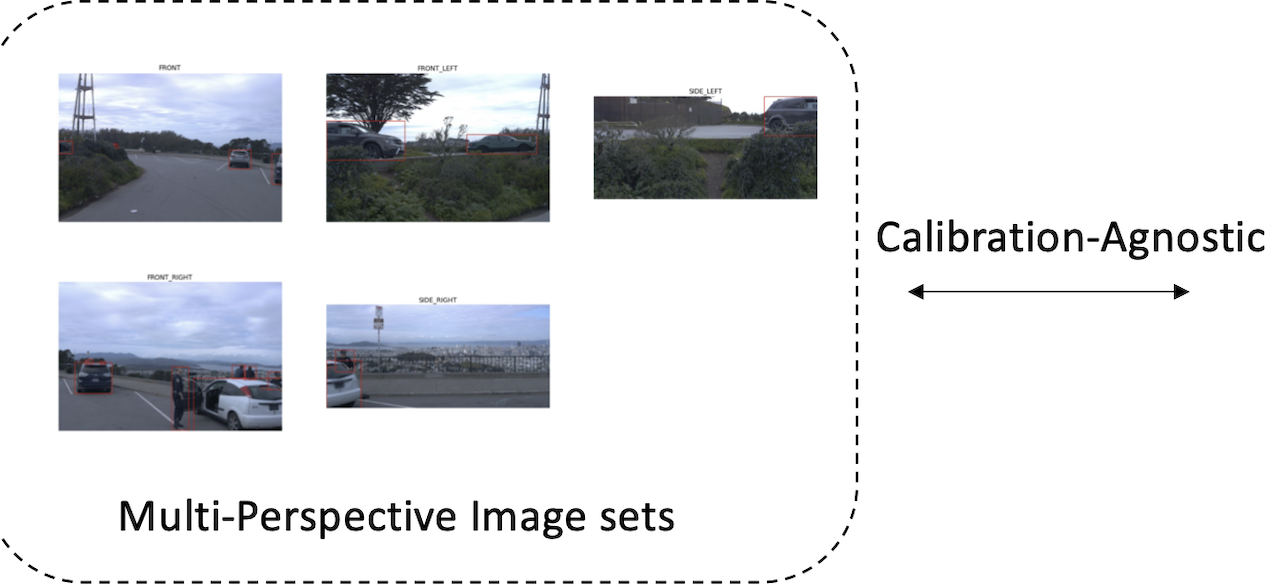
ABOUT THE PROJECT
At a glance
Multi-modal self-supervised pretraining (e.g., CLIP [3]) opens up a new direction for us. CLIP shows superior zero-shot performance with much less image-text paired data (e.g., 12x fewer) than pretraining on the single modality. However, few works explore the multi-modal self-pretraining in 3D perception for autonomous driving. Specifically, self-driving cars mainly exploit cameras and LiDARs as perception sensors, which are complementary to each other. However, the scale of paired image/point-cloud data is limited due to the strict calibration and synchronization.
Therefore, we are motivated to use the non-calibrated image/point-cloud pairs since such data can be much easier to scale-up. Indeed, even without calibration, image and point-cloud in the same scenes capture consistent information which are the keys in the self-supervised learning [1][2][3]. To this end, we propose a calibration-agnostic multi-modal self-supervised pre-training method to achieve label-efficient 3D perception (e.g., using 1% labels to achieve the performance comparable to using 100% labels).
Principal Investigators | researchers | themes |
---|---|---|
Wei Zhan Chenfeng Xu | self-supervised learning, data efficiency, multi-modal 3D perception |