Object-centric Spatial-Temporal Interaction Networks for Rare Event Recognition
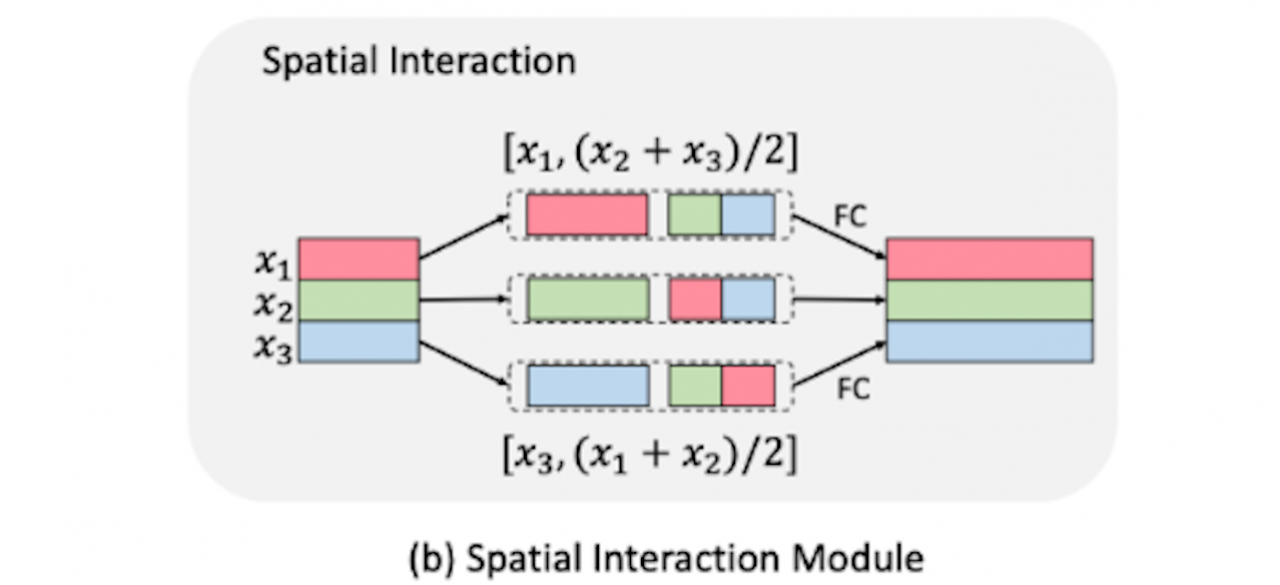
ABOUT THIS PROJECT
At a glance
Events defined by the interaction of objects in a scene are often of critical importance; yet important events may have insufficient labeled examples to train a conventional deep model to generalize to future object appearance. Activity recognition models that represent object interactions explicitly have the potential to learn in a more efficient manner than those that represent scenes with global descriptors. We propose a novel model which can explicitly reason about the geometric relations occurring in the multi-object interactions. We will demonstrate the effectiveness of our models on a driving activities focusing on multi-object interactions with near-collision events. We test the generalization ability of our model in a few-shot learning setting which requires the model to generalize across both object appearance and action category. This is especially crucial in rare event recognition where the labelled data is per definition sparse. We will also explore non-driving applications of our object-based models, such as in human-robot interaction, and in recognition of everyday activities.
PRINCIPAL INVESTIGATORS | RESEARCHERS | THEMES |
---|---|---|
Trevor Darrell | few-shot, activity recognition, object-based models |