Pedestrian Trajectory Prediction Combining Posture and Social Features
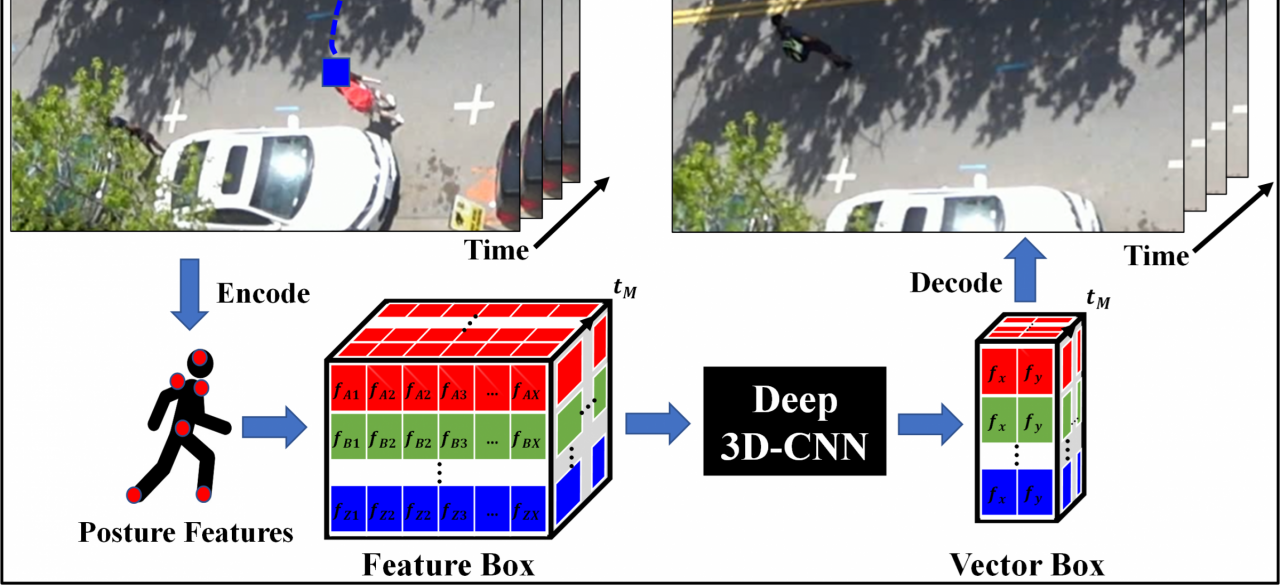
ABOUT THIS PROJECT
At a glance
A primary goal of this study is to explore the combined utilization of social and gesture features based on a machine learning method to predict pedestrian trajectories. The posture and social features present a non-linear relationship, which neural networks are characteristically suitable to deal with. A deep three-dimensional convolutional neural network (3D-CNN), with its non-linear attributes, is an appropriate candidate method to effectively achieve the objective of predicting pedestrian trajectory. The second goal of this work, from a methodological perspective, is to introduce a novel way of using 3D-CNN, which is mostly limited to applications for lidar and camera data processing in the field of autonomous driving. Additionally, we will expand the versatility of OpenPose, which can be used for the extraction of posture features and prediction of future movements. The same methodology can be applied to predicting various agents besides pedestrians, where similar underlying rules of gesture features and social interactions among agents can be estimated using the same concept.
Principal Investigators | researchers | themes |
---|---|---|
I-Hsi Kao | 3D-CNN, image and video processing, trajectory prediction, pedestrian, posture and social features, OpenPose |