Real-Time Perception/ Prediction of Traffic Scene
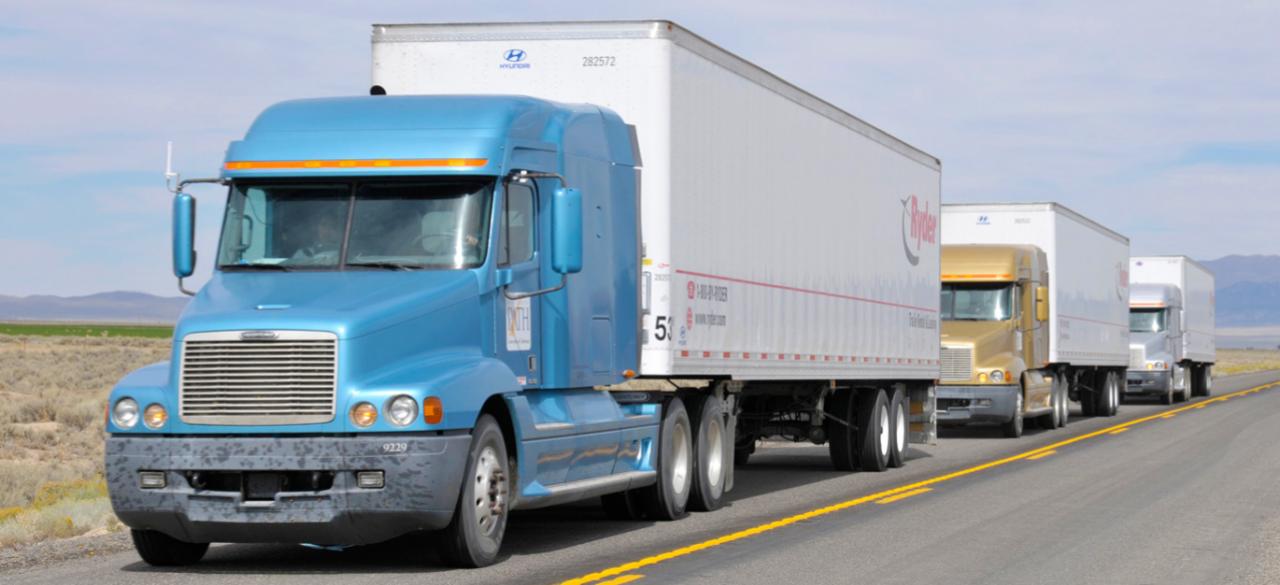
Real-Time Perception/Prediction of Traffic Scene with Deep Learning for Autonomous Driving
At a glance
For automated vehicles driving on public roads, it is necessary for the subject vehicle to accurately determine the traffic environment around it to improve safety and maneuverability of the subject vehicle. The traffic environment includes that of the road geometry, nearby vehicles, and other objects. This information is usually obtained by onboard remote sensors, such as video camera or radar/lidar, and then processed to determine a desired 2D or 3D reference trajectory (position, velocity and acceleration) for feedback control of the subject vehicle. The accuracy of the processed information and determined reference trajectory is critical for the safety and mobility of the vehicle.
Currently, commercial products with sensor fusion of video camera and Doppler radar are available for front target(s) detection/tracking/estimation in real-time. In principle, it could track multiple targets, including vehicles, and provide their distance speed and acceleration -with respect to the subject vehicle. However, only the immediate front target detection/tracking is currently reliable. Detection/tracking of targets in adjacent lanes still needs work to improve its accuracy and reliability.
In this project, the team will assume that real-time traffic scene perception with video camera with respect to the subject vehicle is already available to investigate how to improve the sensor fusion by using more video image data for real-time target detection/tracking/prediction and traffic scene prediction, critical for autonomous vehicles to operate on public roadways. The objectives of the project for the first year are to: collect freeway traffic scene data with space-time synchronized video camera and radar/lidar; fuse video data with radar/lidar data for real-time traffic scene perception; and predict nearby vehicle intention with respect to the subject vehicle using some existing learning algorithm such as stochastic steepest decent with offline learning.
Currently, commercial products with sensor fusion of video camera and Doppler radar are available for front target(s) detection/tracking/estimation in real-time. In principle, it could track multiple targets, including vehicles, and provide their distance speed and acceleration -with respect to the subject vehicle. However, only the immediate front target detection/tracking is currently reliable. Detection/tracking of targets in adjacent lanes still needs work to improve its accuracy and reliability.
In this project, the team will assume that real-time traffic scene perception with video camera with respect to the subject vehicle is already available to investigate how to improve the sensor fusion by using more video image data for real-time target detection/tracking/prediction and traffic scene prediction, critical for autonomous vehicles to operate on public roadways. The objectives of the project for the first year are to: collect freeway traffic scene data with space-time synchronized video camera and radar/lidar; fuse video data with radar/lidar data for real-time traffic scene perception; and predict nearby vehicle intention with respect to the subject vehicle using some existing learning algorithm such as stochastic steepest decent with offline learning.
Principal investigators | researchers | themes |
---|---|---|
Xiao-Yun Lu | Autonomous Vehicles |