Reinforcement Learning for Automated Driving – A Use Case of Ramp Merge Automation
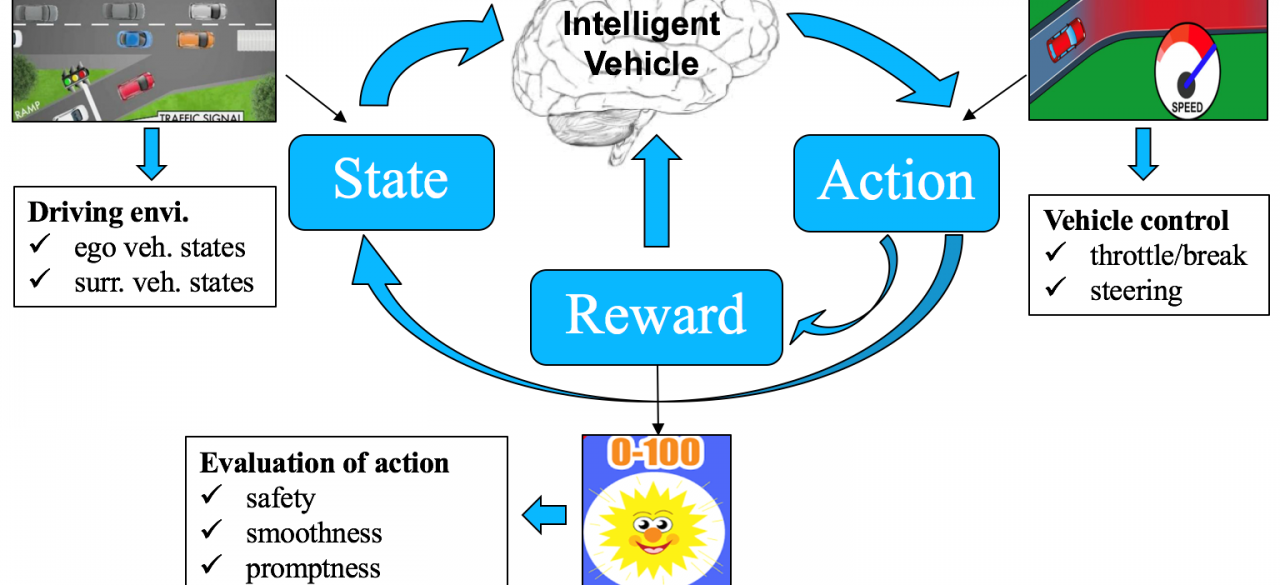
ABOUT THE PROJECT
At a glance
The research work is part of a project, previously titled ‘Supervisory Control in Hybrid Driving Systems - a Use Case of Ramp Merging Automation’, conducted as a core research activity under the Infrastructure project.
Automated freeway auto-pilot systems in developments or those to be introduced to the market are intended for operations on freeways only, but excludes the on-ramp and/or off-ramp automation features. This is mostly due to the consideration that inadequate detection and tracking of other vehicles, geometric and dynamic constraints, as well as uncertainty in traffic movements will present risky conditions for the ego-vehicle. In this study, we focus on the implementation of the automated ramp-merge use case based on a machine-learning method.
To tackle the complicated ramp merging problem, we apply Reinforcement Learning (RL) for finding an optimal driving policy for merging vehicles. We formulate a long-term reward which represents the expected effect of the current action on the future objective. The reward function includes several components: (1) safety, represented by spacing to preceding and following vehicles when merge maneuver is in process, (2) smoothness, by accounting the acceleration levels experienced by the ego-vehicle, (3) efficiency, represented by time (or inverse of speed) needed to complete the merge action. Besides, we treat the problem in an interactive driving environment, that is, we take into consideration the interaction of the ego vehicle (ramp merge vehicle) with its surrounding vehicles.
We test the developed ramp merge automation model under simulation platform that was developed to generate diverse traffic conditions. In the simulation models, car-following controllers are devised and assigned to all other vehicles so that they behave in a realistic manner. Our preliminary results show that the automated merge actions can be executed safely, smoothly and promptly under the interactive driving environment on the mainline.
PRINCIPAL INVESTIGATORS | RESEARCHERS | THEMES |
---|---|---|
Pin Wang | Driver-Machine Interaction |