Representation Learning for Person Segmentation and Tracking
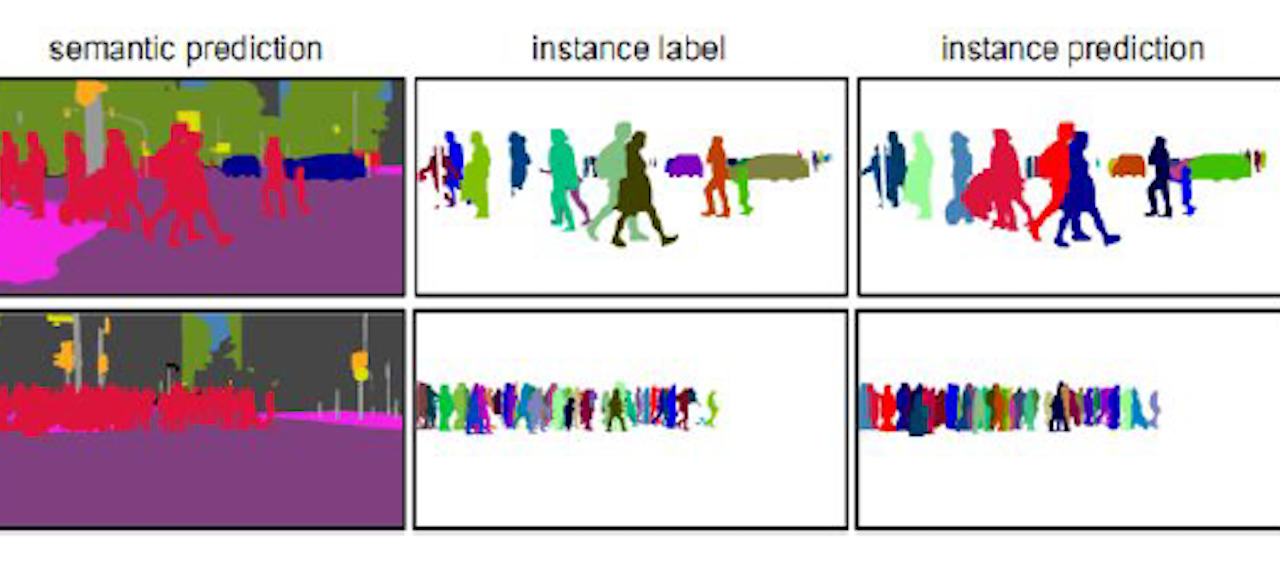
ABOUT THIS PROJECT
At a glance
To understand human activities and behaviour patterns from videos, we need to extract a visual representation that captures information relevant for individual persons in a crowd, ideally from naturally captured data with minimal annotations. We propose to leverage our expertise on representation learning for image classification, open long-tailed recognition, object detection, semantic and instance segmentation, and extend it to representation learning of persons from videos, with partial or no human annotations at all on the video frames, for the sake of accurately segmenting and tracking individual persons over time, at the same time facilitating the discovery of behaviour patterns.
We consider an overhead surveillance application in an attendant-less store. These images have scene coverage but skewed and occluded views of individual persons. Crowded crowds, staggered movements, person wearing dark clothing, person squatting down, could all pose additional challenges on segmenting and tracking individuals accurately without mix-ups. On the other hand, there could be additional measurements from shelves, such as infrared ray readings, weight meters, and camera images. These could be exploited during or after visual representation learning. Our goal is to infer "Who is doing what to the store?" more accurately, so that events such as whether an item is moved or taken away and by whom etc can be analyzed.
Principal investigators | researchers | themes |
---|---|---|
Long Lian Runtao Liu Xudong Wang Zhirong Wu | representation learning from videos, weakly supervised learning, unsupervised learning, person segmentation, person tracking, activity recognition, event detection |