Scene Understanding and Forecasting in Contextual Environment – From Pixel to Semantic
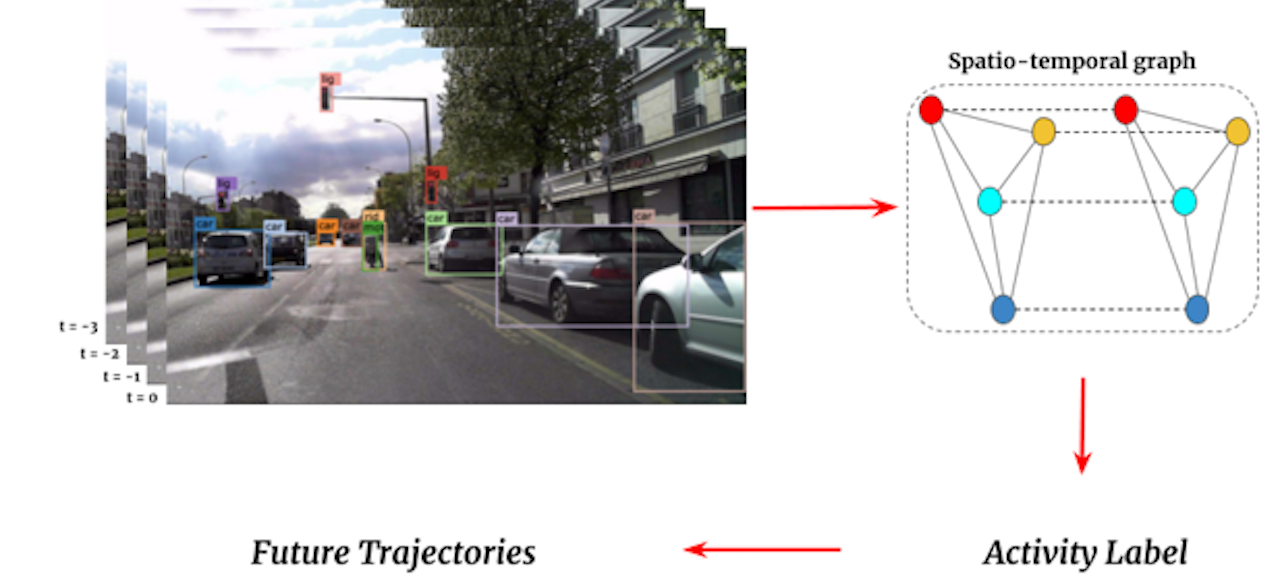
ABOUT THIS PROJECT
At a glance
The lack of capability to properly recognize the situation and foresee the need to change course is a severe shortcoming in current automated driving systems.
The variety of objects and scenes that were involved in real-world crashes revealed a broader problem, i.e., it is not about a single type of scenes that the system fails to understand. To overcome this shortcoming, the effective solution lies in a more perceptive system that must recognize and anticipate the future paths of all objects in the driving scene semantically. Addressing these challenges, we propose to focus on two fundamental issues: (1) how to correctly recognize the unusual/abnormal event in the driving scene; (2) how to properly foresee the behavior and trajectories of relevant objects under these situations. The goal of this project is to define a framework that enables the scene understanding of the driving environment, so that a highly automated driving system can recognize hazards and respond accordingly.
principal investigators | researchers | themes |
---|---|---|
Yuke Li | Scene Understanding, Forecast in Context, From Pixel to Semantic Interpretation, Automated Driving, Graph Model, Few Shot Learning |