Self-supervised Visual Pre-training for Motor Control
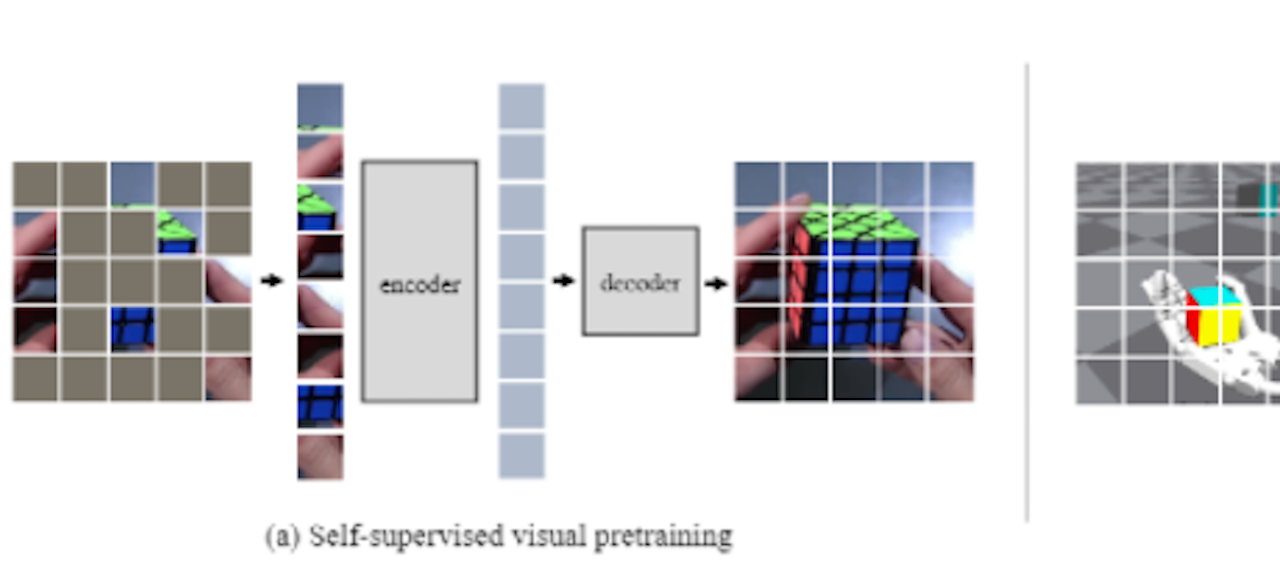
ABOUT THE PROJECT
At a glance
We show that self-supervised visual pretraining from real-world images is effective for learning complex motor control tasks from pixels.We train the visual representation by masked modeling of natural images. We then integrate neural network controllers with reinforcement learning on the frozen representations. We do not perform any task-specific fine-tuning of the encoder;the same representations are used for all motor control tasks. To accelerate progress in learning from pixels, we contribute a benchmark suite of hand-designed tasks varying in movement types,scenes, and robots. To the best of our knowledge,ours is the first self-supervised model to exploit natural images at scale for motor control. With-out relying on labels, state-estimation, or expert demonstrations, our approach consistently outper-forms supervised baselines by up to 80% absolute success rate, sometimes even matches the oracle state performance. We also find that the choice of visual data plays an important role; e.g., images of human-object interactions work well for manip-ulation tasks. Finally, we show that our approach generalizes across robots, scenes, and objects. Our effort will release models and evaluations.
principal investigators | researchers | themes |
---|---|---|
Self-supervised, Pre-training, Motor Control |