Simultaneous On-Target Domain Adaptation and Performance Prediction
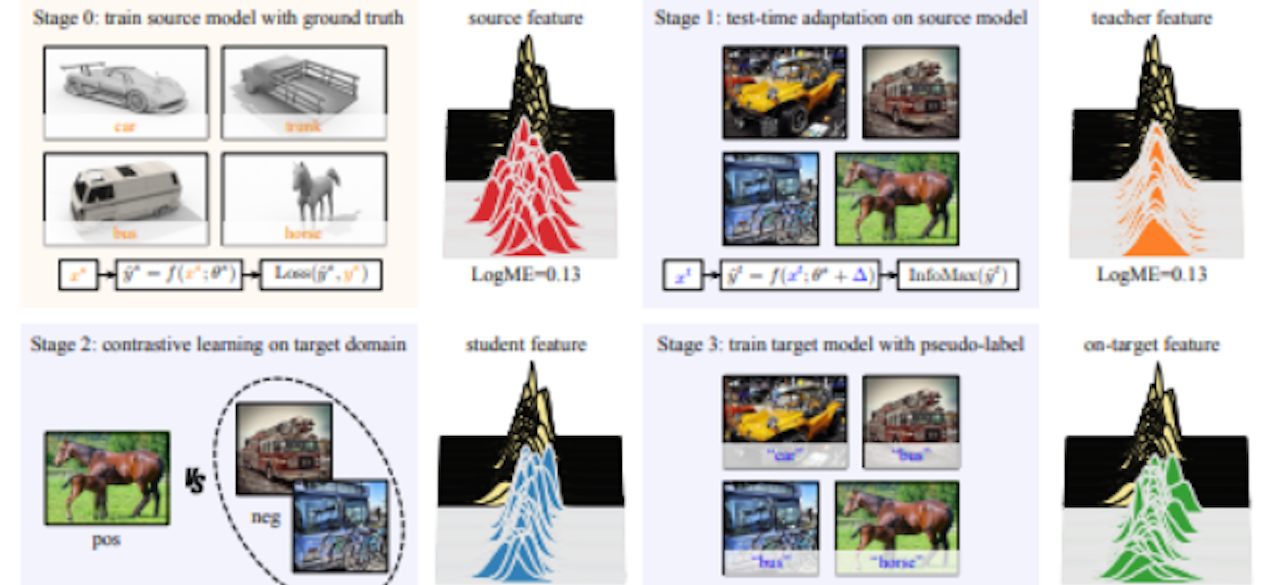
ABOUT THE PROJECT
At a glance
Building machine learning models that perform reliably in a wide range of environments is a central challenge for machine learning researchers. Research on the problem of unsupervised domain adaptation aims to develop approaches which improve a model’s performance in new domains by means of adaptation. While work on automatic model evaluation attempts to estimate a model’s accuracy over unlabeled test data from a new domain. However, predicting a model’s accuracy after adapting the model to a new domain comes with a new set of challenges. Being able to reliably improve a model’s performance in a novel domain and accurately estimate its real accuracy is an important step towards developing more reliable machine learning systems. We have developed a method for performance prediction that is practical and scalable, and is summarized in the following figures.
principal investigators | researchers | themes |
---|---|---|
Trevor Darrell | Domain Adaptation, Performance Prediction, Self-supervised learning |