Spatio-temporal 3D reconstruction of Pedestrians, Objects, and the Environment from Self-Driving Data
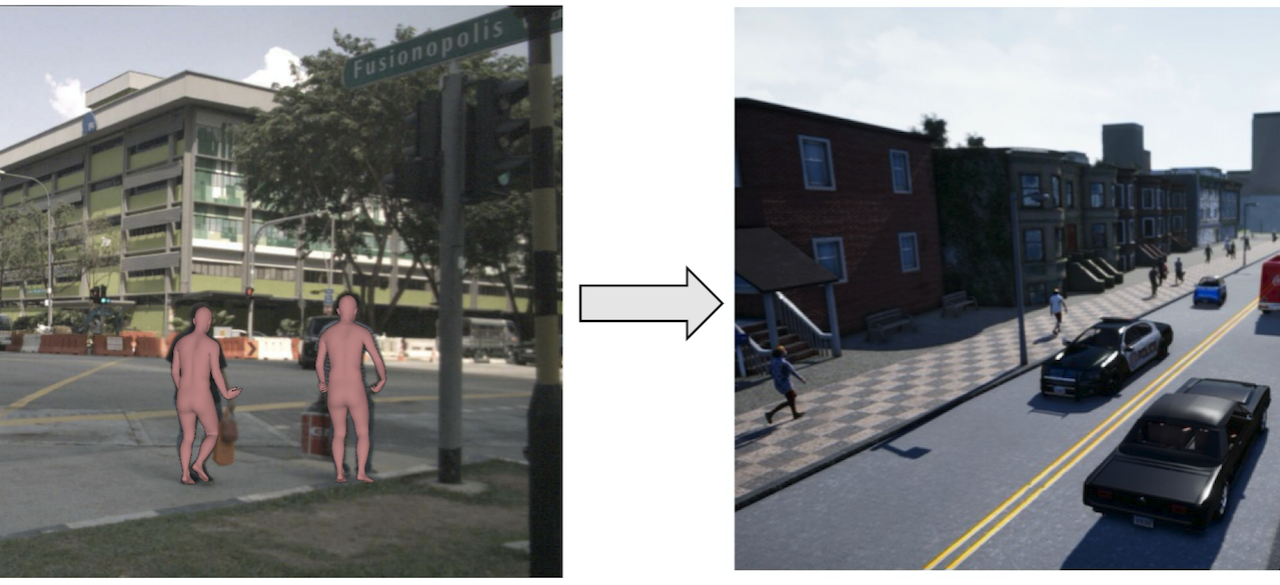
ABOUT THIS PROJECT
At a glance
Understanding pedestrian behavior in self driving requires 3D analysis of the pedestrian in question, but also the 3D location and pose of other pedestrians and objects, such as cars, vegetation and the road, in the scene. Yet, today’s 3D human mesh recovery techniques mostly focus on the 3D reconstruction of a single person in isolation. In this work, we will propose an approach that can reconstruct the entire scene in 3D, including the people, objects, and the environment from self-driving data over time. This approach will incorporate the 3D context (road, objects, other people) in order to recover a physically consistent dynamic 3D scene reconstruction via utilizing the LiDAR data. Resulting 3D reconstructions of humans and objects will be useful for multitude of applications such as pedestrian path prediction, data simulation, and learning a data-driven interaction prior between humans and objects that can be used for monocular image analysis.
principal investigators | researchers | themes |
---|---|---|
Angjoo Kanazawa | Computer Vision, 3D Reconstruction, 3D Human Understanding, 3D Vision, 3D dataset |
This project is a continuation of: "Predicting Pedestrian Behavior from 3D Cues".