Towards safe self-driving by reinforcement learning with maximization of diversity of future options
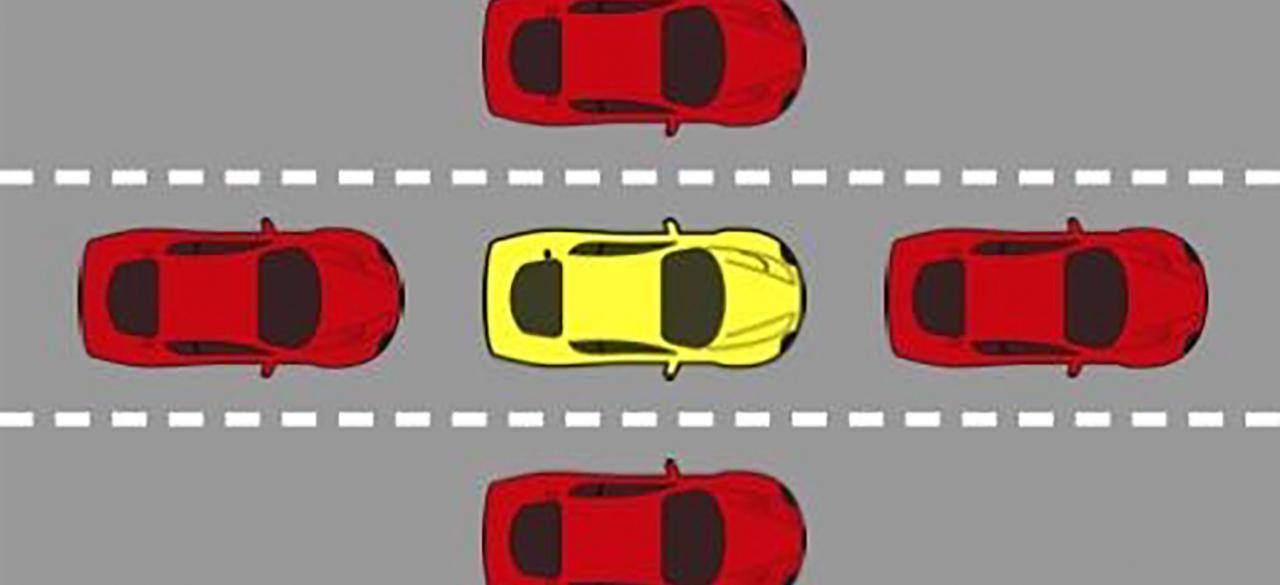
ABOUT THE PROJECT
At a glance
Motivation: The ultimate goal of driving is to arrive at a destination fast and safe. So, driving is a trade-off between speed and safety. An extremely safe driver may have troubles with changing a line, with merging onto a highway, and with other driving tasks that involve risks. On another hand, an extremely speedy driver is prone to car accidents. A good driver balances these two objectives. In this work we formalize this trade-off by combining reinforcement learning for global planning with an information theoretic approach for local estimation of safety.
Background: Reinforcement learning (RL) is a well-established framework for planning with predefined rewards. Recently, deep neural networks were successfully applied to a number of driving tasks. However, there is still no consensus about an operational definition of safety in driving. Safety is a challenging concept, comprising of a) careful actions by a driver and b) avoidance of situations when other drivers may involve a driver in a car accident. We argue that safety in driving can be characterized in general by the diversity of future options that are available for a driver. Strictly speaking, the more diverse future is, the safer a particular state is. Recently, an information theoretic (IT) approach was suggested for estimation of the diversity of future options, which also generalizes the concept of controllability in dynamical systems. The following traffic scenarios demonstrate the usefulness of the diversity of future options for safety, and the insufficiency of RL to guarantee safe driving. Dangerous trap avoidance: The driver in the yellow car (in the figure below) wants to arrive at home as fast as possible. At some point of time they get trapped by the four red cars, driving even faster than they usually do. The only action that Alex can take is to follow the red cars, which is also the optimal action from the viewpoint of RL. However, this situation is very unsafe for Alex, because a wrong action by any one of the red cars will inevitably involve Alex in a car accident. Alex can avoid this dangerous situation by estimating ahead a number of future options she would have before entering the trap. The ability of
future diversity to predict and escape traps was previously reported in. Collision prevention: the diversity of future states reduces dramatically when an agent meets an object that it can not manipulate controllably. This is due to the fact that immutable objects reduces the number of future options. As shown in, intrinsically motivated agents prefer to avoid collisions by moving to states with a larger controllable space. In the above scenarios, car accidents can be prevented by balancing the objective to arrive at a destination fast with the objective to keep future options open, which addresses safety.
principal investigators | researchers | themes |
---|---|---|
Pieter Abbeel | self-driving, safety, reinforcement learning, intrinsic motivation, deep learning |