Uncertainty-Aware Reinforcement Learning for Interaction-Intensive Driving Tasks
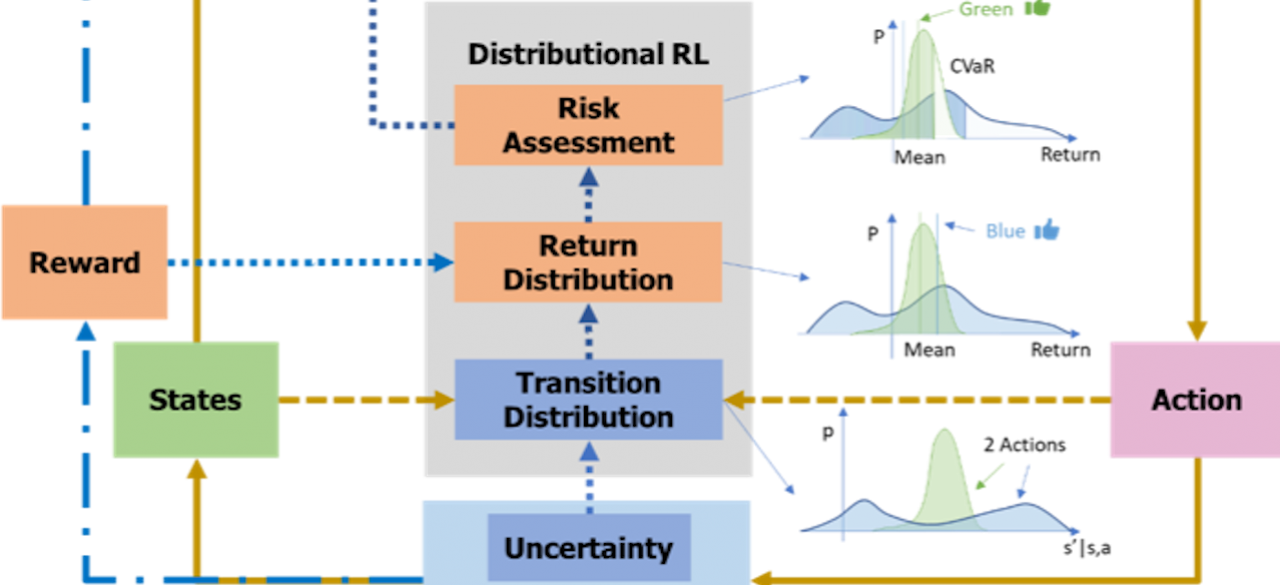
ABOUT THIS PROJECT
At a glance
In daily driving, drivers often need to deal with various types of uncertainties, among which the intrinsic uncertainty of road users’ behaviors is a critical factor. This type of uncertainty is called aleatoric. Another type of uncertainty is epistemic, which is related to knowledge gaps of a model. These uncertainties directly impact how the ego vehicle interacts with other road users and affect how drivers make decisions at the tactical level. It is important to incorporate such uncertainties into the driving strategies to ensure safety.
In this project, we propose to develop uncertainty-aware decision-making algorithms for interaction-intensive driving tasks, e.g. performing maneuvers in dense traffic and negotiating with surrounding vehicles with highly variant behaviors. We will exploit and improve current RL methods to address aleatoric (with distributional RL related methods) and epistemic uncertainties (with Bayesian related methods and bootstrapping and dropout techniques).
principal investigators | researchers | themes |
---|---|---|
Pin Wang | Uncertainty-aware Decision-making, Interaction-intensive Driving, Reinforcement Learning, Bayesian Methods, Aleatoric and Epistemic Uncertainties |