Vehicle Dynamic Estimation based on Image Sensor & Radar Fusion
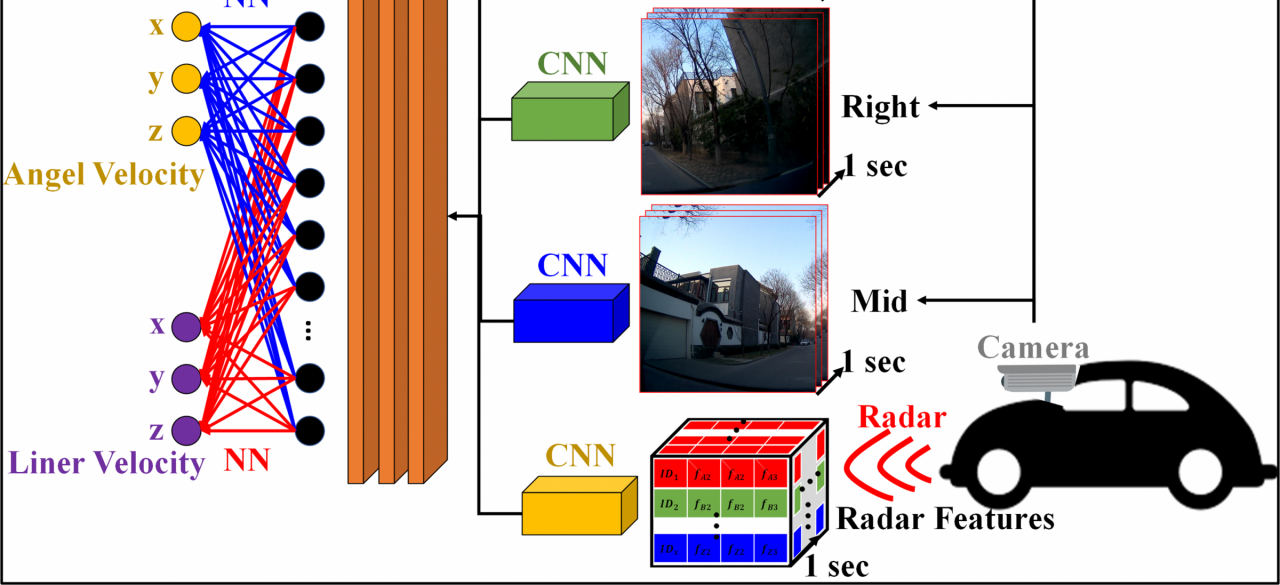
ABOUT THIS PROJECT
At a glance
This project is aimed at the development of an intelligent vehicle dynamics estimation model based on a camera-radar fusion system. This model does not count on the conventional data sources of IMU, steering angle, or wheel speed. Instead, our model will use a deep learning approach for feature extraction and integration of multiple data sources.
Furthermore, we expect the methodology developed in this project can be extended to other vehicle applications. As an example, temperature, pressure, rotational speed, casing vibration, control actuator positions, flow rate, clearance between stationary and rotating parts, and lubrication oil quality can be correlated with engine health. However, it takes a great deal of time and energy to perform simulation calculations using inverted mathematics models. If a multi-feature extraction and regression approach is taken, reducing human resources can be achieved. As another example, the health status of the driver can also be judged. Contact and capacitive electrodes capture electrocardiography, and heart rate and heart rate variability are computed from the recordings. Cameras provide video data, from which remote photoplethysmography is generated. Using a variety of human characteristics can determine the driver's excitement, fatigue, and other parameters.
The same model design concept can establish correlations between various signals. Thus, the multi-feature processing model has a wide range of applications. The general goal of this project is to create a deep learning model with a methodology suitable for big data with multi-sensor features.
principal investigators | researchers | themes |
---|---|---|
Ching-Yao Chan | I-Hsi Kao | sensor fusion, radar and camera, vehicle dynamics estimation, CNN, Multi-feature data processing, deep learning |