Safe and Effective Learning and Control through Formal Simulation
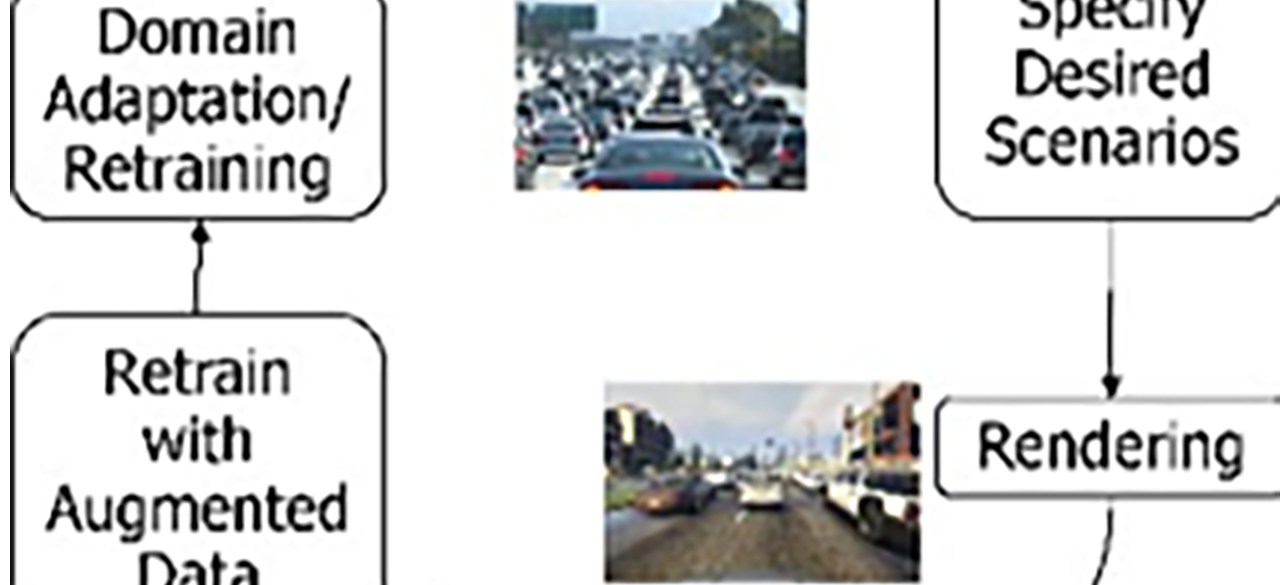
ABOUT THE PROJECT
At a glance
The goal of the proposed project is to leverage existing simulation environments more effectively for safe and accurate learning by using formal methods -- an approach we term formal simulation. We aim to achieve this goal by continuing our approach of starting with real-world data, creating scenarios in a programmatic fashion to drive simulation, use formal methods to systematically search for unsafe and other interesting cases in simulation, yielding new data which is then used to augment existing datasets and retrain machine learning components and/or redesign controllers. We will integrate this effort with domain adaptation to close the loop between simulation and the real world.
To see work built on results from this project, see "Safe and Effective Perception and Control through Formal Simulation".
Principal investigators | researchers | themes |
---|---|---|
Sanjit Seshia | deep neural networks, simulation, verification, control, testing, training, data generation/augmentation |